In this chapter, we introduced different architectures for recurrent neural networks, and pointed out some of their limitations and capabilities. By introducing a naive Markovian model, we compared the efficiency of introducing such complicated architectures. When applied to the text generation problem, we saw that these different architectures had a noticeable improvement in the quality of the predictions. For training networks, we introduced different methods. The classical backpropagation algorithm and other gradient-free methods that are useful to solve black-box optimization problems.




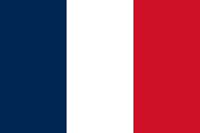


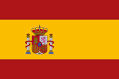



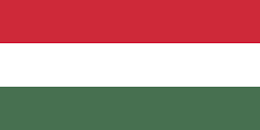

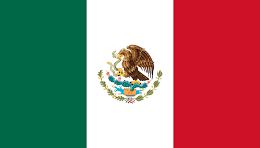


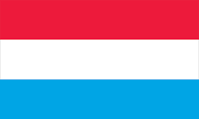



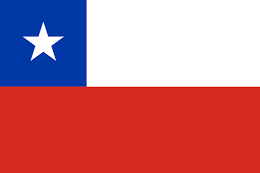


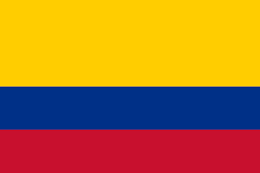
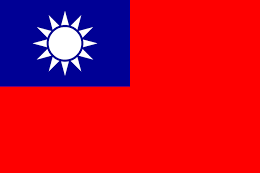


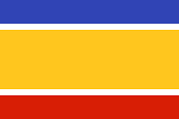
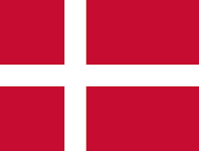
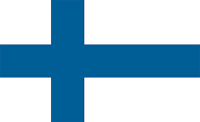



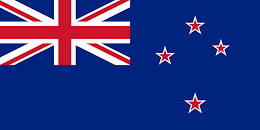





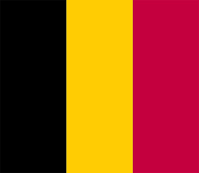

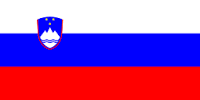




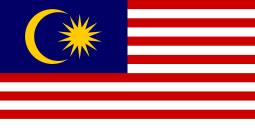


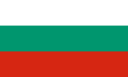


