In this chapter, we learned how the values of various actions in a given state are calculated. We then learned how the agent updates the Q-table using the discounted value of taking an action in a given state. In the process of doing this, we learned how the Q-table is infeasible in a scenario where the number of states is high. We also learned how to leverage deep Q-networks to address the scenario where the number of possible states is high. Next, we moved on to leveraging CNN-based neural networks while building an agent that learned how to play Pong using DQN based on fixed targets. Finally, we learned how to leverage DQN with fixed targets to perform self-driving using the CARLA simulator. As we have seen repeatedly in this chapter, you can use deep Q-learning to learn very different tasks – such as CartPole balancing, playing Pong, and self-driving navigation – with almost the same code. While this is not the end of our journey into exploring RL, at this point...




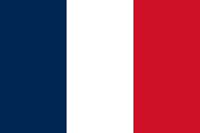


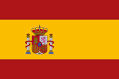



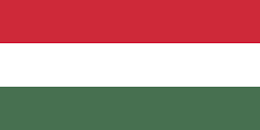

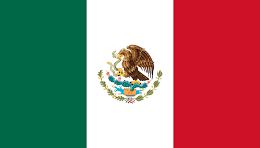


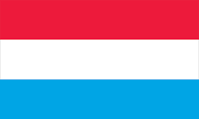



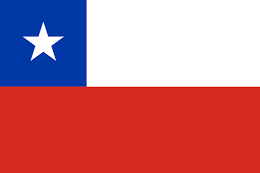


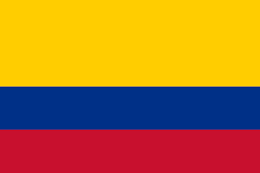
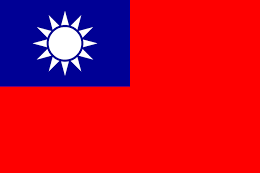


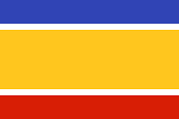
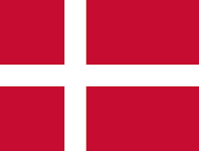
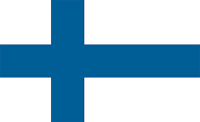



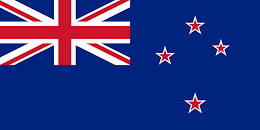





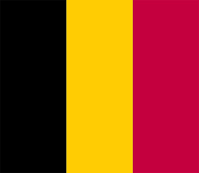

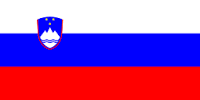




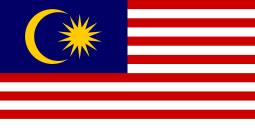


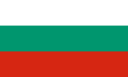


