Thompson (Posterior) sampling
The goal in MAB problems is to estimate the parameter(s) of the reward distribution for each arm (that is ad to display in the above example). In addition, measuring our uncertainty about our estimate is a good way to guide the exploration strategy. This problem very much fits into the Bayesian inference framework, which is what Thompson sampling leverages. Bayesian inference starts with a prior probability distribution, an initial idea, for the parameter , and updates this prior as data becomes available. Here,
refers to the mean and variance for a normal distribution, and to the probability of observing a "1" for Bernoulli distribution. So, the Bayesian approach treats the parameter as a random variable given the data.
The formula for this is given by:
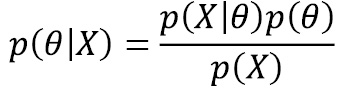
In this formula, is the prior distribution of
, which represents the current hypothesis on its distribution.
represents the data, with which we obtain a posterior...