Keras (https://keras.io/) is an open source, high-level neural network API written in Python (compatible with Python 2.7-3.6). It is capable of running on top of TensorFlow, Microsoft Cognitive Toolkit, Theano, or PlaidML, and was developed with a focus on enabling fast experimentation. In this section, we are going to see two examples. In the first example, we are going to see how to solve a linear regression problem using the same input data as the TensorFlow example in the previous section. In the second example, we will classify some handwritten digits using the MNIST dataset in the same way we also performed in the previous section with TensorFlow. This way, you can clearly see the differences between the two libraries when solving the same kind of problems.




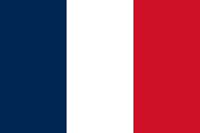


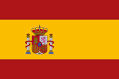



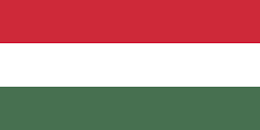

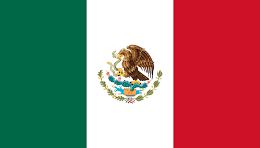


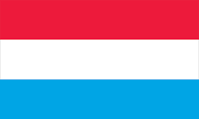



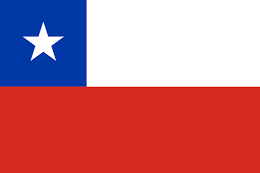


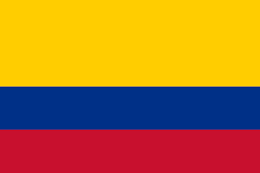
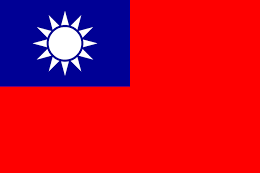


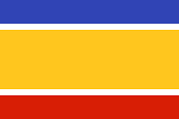
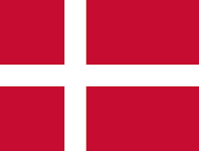
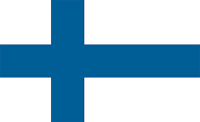



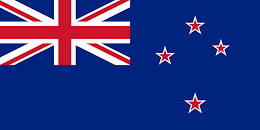





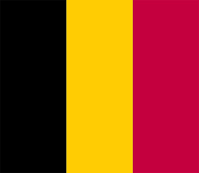

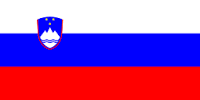




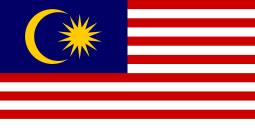


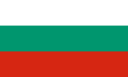


