A Graph Neural Network (GNN) extends CNN learning to graph data. A graph can be represented as a combination of nodes and edges, where nodes represent the features of the graph and edges joins adjacent nodes, as shown in the following image:
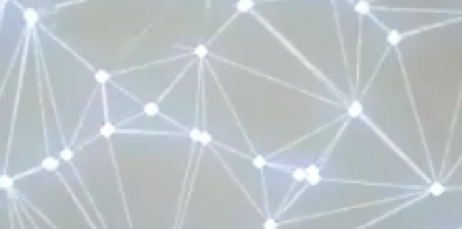
In this image, the nodes are illustrated by solid white points and the edges by the lines joining the points.
The following equations describe the key parameters of the graph:
The transformations of the graph into a vector consisting of nodes, edges, and the relationships between the nodes are called the graph embeddings. The embeddings vector can be represented by the following equation:


The following list describes the elements of the preceding equation:
- h[n] = State embedding for current node n
- hne[n] = State embedding of the neighborhood of the node n
- x[n] = Feature of the node n
- xe[n] = Feature of the edge of...