In this chapter, we have explored the practical applications of AI, data science, machine learning, deep learning, and causal inference. We have defined machine learning as a field that studies algorithms that use data to support decisions and give insights without specific instructions. There are three main machine learning methodologies: supervised, unsupervised, and reinforcement learning. In practice, the most common types of task we solve using machine learning are regression and classification. Next, we described deep learning as a subset of machine learning devoted to studying neural network algorithms. The main application domains of deep learning are computer vision and NLP. We have also touched on the important topic of causal inference: the field that studies a set of methods for discovering causal relationships in data. You now know a lot about general data...




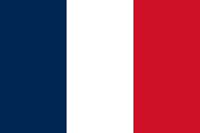


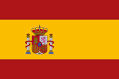



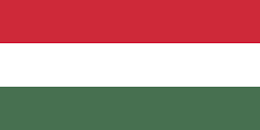

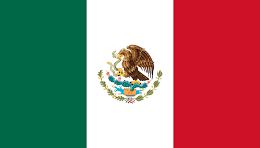


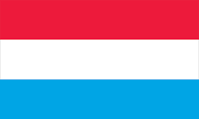



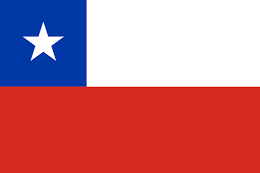


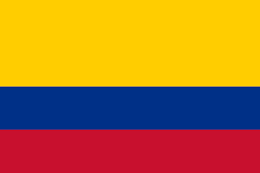
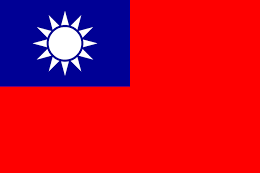


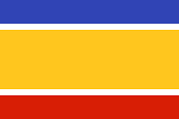
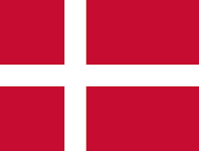
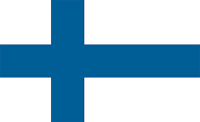



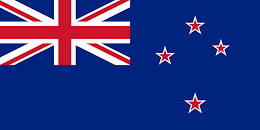





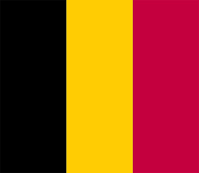

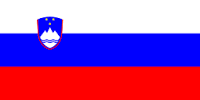




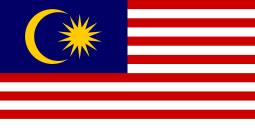


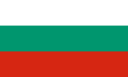


