To recognize different types of motion activities, we will train the KNN classifier. The idea of the method is to find k training samples closest to the sample with an unknown label, and predict the label as a most frequent class among those k. That's it:
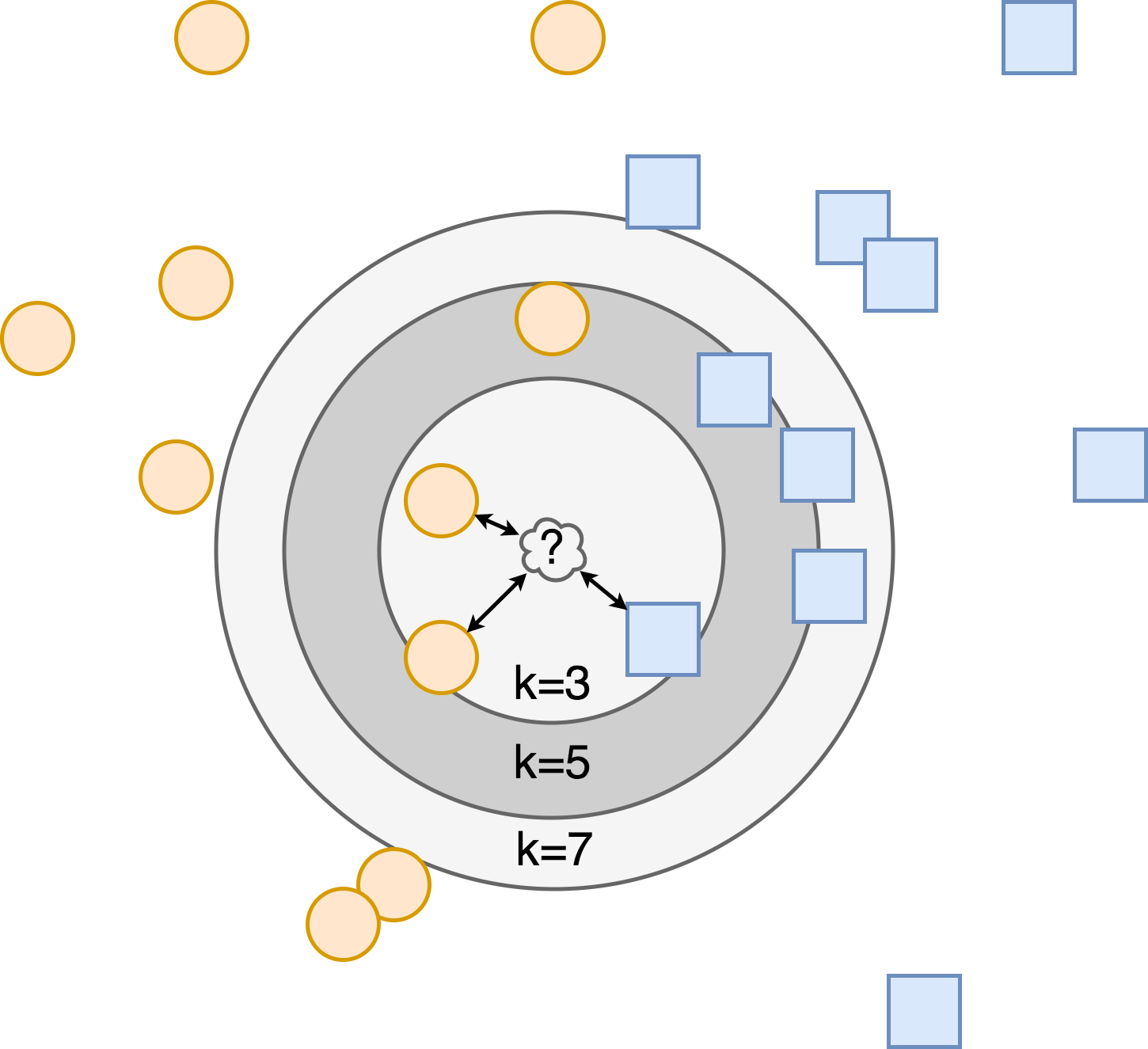
Figure 3.5: KNN classification algorithm. The new data point marked with ? gets classified based on the classes of its neighbors.
Note how the choice of neighbor number affects the result of classification.
In fact, the algorithm is so simple, that it's tempting to formulate it in more complicated terms. Let's do it. The secret sauce of a KNN is a distance metric: function, which defines how close to each other two samples are. We have discussed several of them already: Euclidean, Manhattan, Minkowski, edit distance, and DTW. Following the terminology, samples are points in...