There are numerous real-world use cases where the number of features available that may potentially be used to train a model is very large. A common example is economic data, and using its constituent stock price data, employment data, banking data, industrial data, and housing data together to predict the gross domestic product (GDP). Such types of data are said to have high dimensionality. Though they offer numerous features that can be used to model a given use case, high-dimensional datasets increase the computational complexity of machine learning algorithms, and more importantly may also result in over fitting. Over fitting is one of the results of the curse of dimensionality, which formally describes the problem of analyzing data in high-dimensional spaces (which means that the data may contain many attributes, typically hundreds or even thousands...




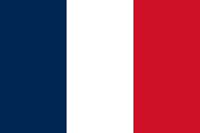


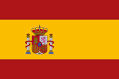



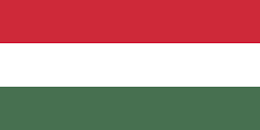

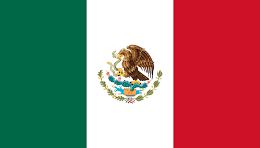


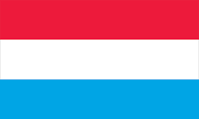



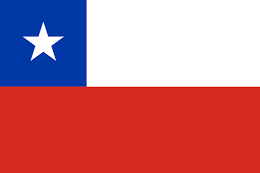


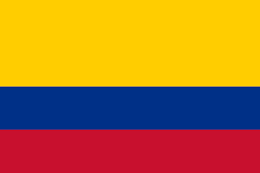
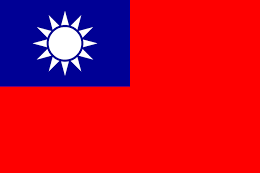


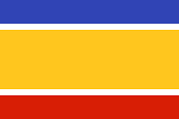
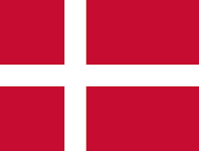
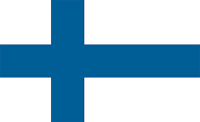



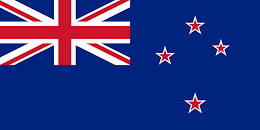





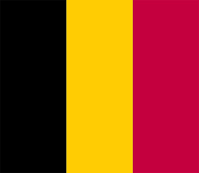

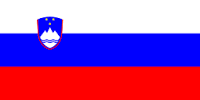




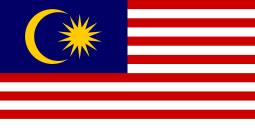


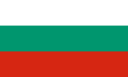


