TensorFlow Privacy (https://github.com/tensorflow/privacy) is a relatively new addition to the TensorFlow family. This Python library includes implementations of TensorFlow optimizers for training machine learning models with differential privacy. A model that has been trained to be differentially private does not non-trivially change as a result of the removal of any single training instance from its dataset. (Approximate) differential privacy is quantified using epsilon and delta, which give a measure of how sensitive the model is to a change in a single training example. Using the Privacy library is as simple as wrapping the familiar optimizers (for example, RMSprop, Adam, and SGD) to convert them to a differentially private version. This library also provides convenient tools for measuring the privacy guarantees, epsilon,...




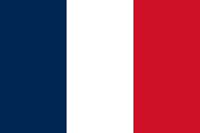


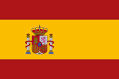



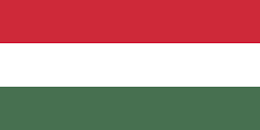

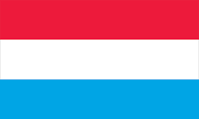





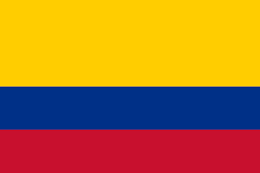
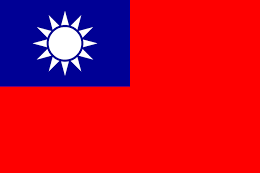
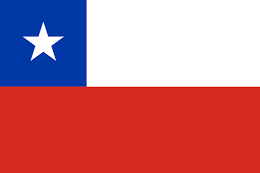



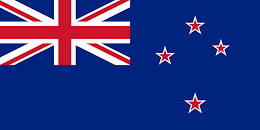
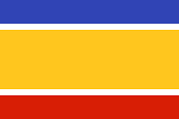
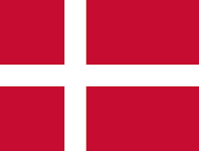
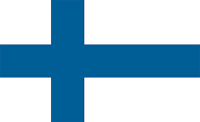







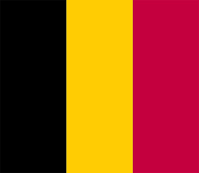

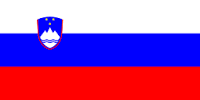





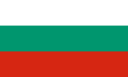


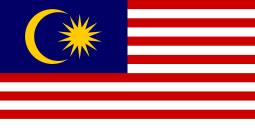



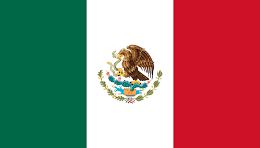
