This chapter begins by analyzing linear classification problems, with particular focus on logistic regression (despite its name, it's a classification algorithm) and stochastic gradient descent approaches. Even if these strategies appear too simple, they're still the main choices in many classification tasks. Speaking of which, it's useful to remember a very important philosophical principle: Occam's razor. In our context, it states that the first choice must always be the simplest and only if it doesn't fit, it's necessary to move on to more complex models. In the second part of the chapter, we're going to discuss some common metrics useful to evaluate a classification task. They are not limited to linear models, so we use them when talking about different strategies as well.




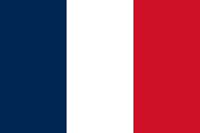


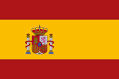



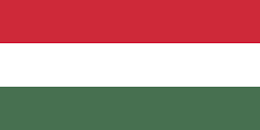

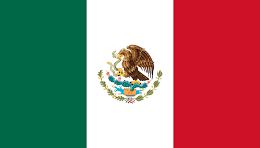


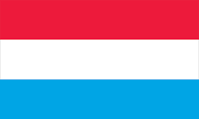



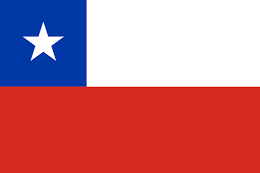


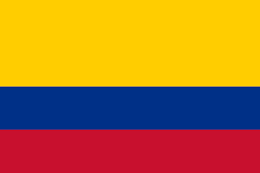
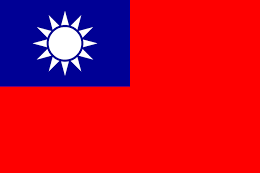


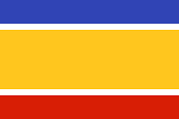
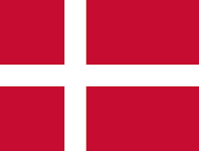
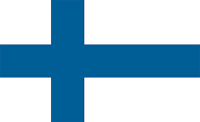



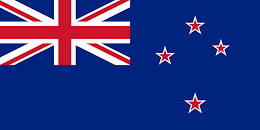





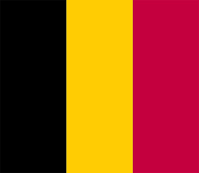

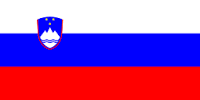




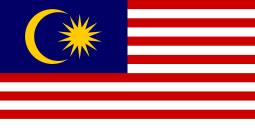


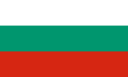


