Although there is no specific batch size or learning rate that works for all models, we can find the best values for them by experimenting with multiple training instances. The primary step is to experiment with a set of batch size values and learning rates with the model. Observe the efficiency of the model by evaluating additional parameters such as Precision, Recall, and F1 Score. Test scores alone don't confirm the model's performance. Also, parameters such as Precision, Recall, and F1 Score vary according to the use case. You need to analyze your problem statement to get an idea about this. In this recipe, we will walk through key steps to determine the right batch size and learning rates.
Determining the right batch size and learning rates
How to do it...
- Run the training instance multiple times and track the evaluation metrics.
- Run experiments by increasing the learning rate and track the results.
How it works...
Consider the following experiments to illustrate step 1.
The following training was performed on 10,000 records with a batch size of 8 and a learning rate of 0.008:
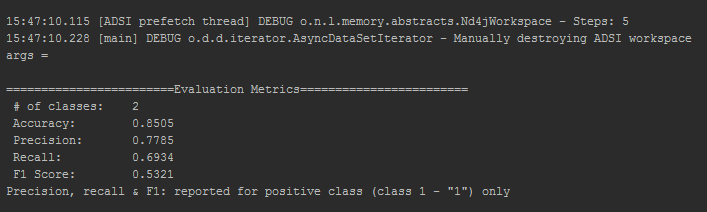
The following is the evaluation performed on the same dataset for a batch size of 50 and a learning rate of 0.008:
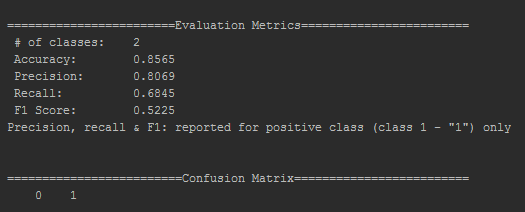
To perform step 2, we increased the learning rate to 0.6, to observe the results. Note that a learning rate beyond a certain limit will not help efficiency in any way. Our job is to find that limit:
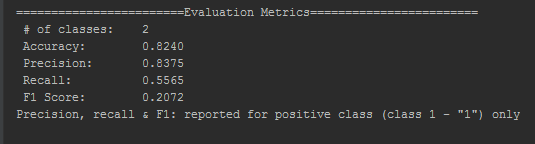
You can observe that Accuracy is reduced to 82.40% and F1 Score is reduced to 20.7%. This indicates that F1 Score might be the evaluation parameter to be accounted for in this model. This is not true for all models, and we reach this conclusion after experimenting with a couple of batch sizes and learning rates. In a nutshell, you have to repeat the same process for your model's training and choose arbitrary values that yield the best results.
There's more...
When we increase the batch size, the number of iterations will eventually reduce, hence the number of evaluations will also be reduced. This can overfit the data for a large batch size. A batch size of 1 is as useless as a batch size based on an entire dataset. So, you need to experiment with values starting from a safe arbitrary point.
A very small learning rate will lead to a very small convergence rate to the target. This can also impact the training time. If the learning rate is very large, this will cause divergent behavior in the model. We need to increase the learning rate until we observe the evaluation metrics getting better. There is an implementation of a cyclic learning rate in the fast.ai and Keras libraries; however, a cyclic learning rate is not implemented in DL4J.