Data transformation alone may not improve the neural network's efficiency. The existence of large and small ranges of values within the same dataset can lead to overfitting (the model captures noise rather than signals). To avoid these situations, we normalize the dataset, and there are multiple DL4J implementations to do this. The normalization process converts and fits the raw time series data into a definite value range, for example, (0, 1). This will help the neural network process the data with less computational effort. We also discussed normalization in previous chapters, showing that it will reduce favoritism toward any specific label in the dataset while training a neural network.




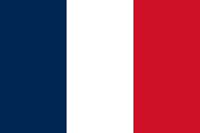


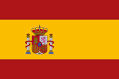



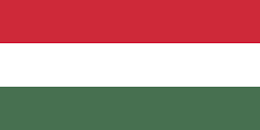

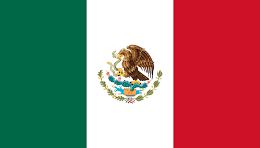


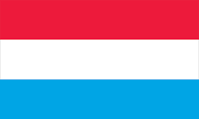



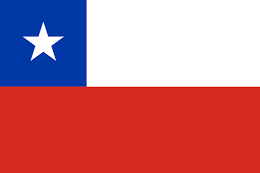


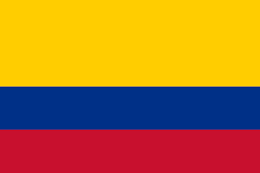
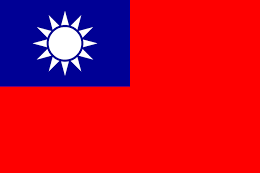


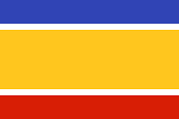
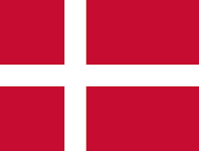
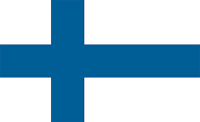



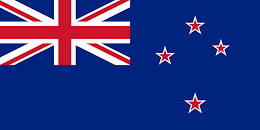





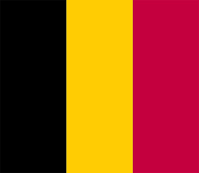

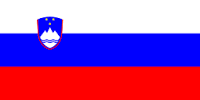




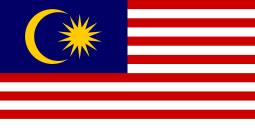


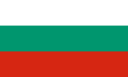


