Camera calibration with OpenCV
In this section, you will learn how to take objects with a known pattern and use them to correct lens distortion using OpenCV.
Remember the lens distortion we talked about in the previous section? You need to correct this to ensure you accurately locate where objects are relative to your vehicle. It does you no good to see an object if you don't know whether it is in front of you or next to you. Even good lenses can distort the image, and this is particularly true for wide-angle lenses. Luckily, OpenCV provides a mechanism to detect this distortion and correct it!
The idea is to take pictures of a chessboard, so OpenCV can use this high-contrast pattern to detect the position of the points and compute the distortion based on the difference between the expected image and the recorded one.
You need to provide several pictures at different orientations. It might take some experiments to find a good set of pictures, but 10 to 20 images should be enough. If you use a printed chessboard, take care to have the paper as flat as possible so as to not compromise the measurements:

Figure 1.20 – Some examples of pictures that can be used for calibration
As you can see, the central image clearly shows some barrel distortion.
Distortion detection
OpenCV tries to map a series of three-dimensional points to the two-dimensional coordinates of the camera. OpenCV will then use this information to correct the distortion.
The first thing to do is to initialize some structures:
image_points = [] # 2D points object_points = [] # 3D points coords = np.zeros((1, nX * nY, 3), np.float32)coords[0,:,:2] = np.mgrid[0:nY, 0:nX].T.reshape(-1, 2)
Please note nX
and nY
, which are the number of points to find in the chessboard on the x
and y
axes,, respectively. In practice, this is the number of squares minus 1.
Then, we need to call findChessboardCorners()
:
found, corners = cv2.findChessboardCorners(image, (nY, nX), None)
found
is true if OpenCV found the points, and corners
will contain the points found.
In our code, we will assume that the image has been converted into grayscale, but you can calibrate using an RGB picture as well.
OpenCV provides a nice image depicting the corners found, ensuring that the algorithm is working properly:
out = cv2.drawChessboardCorners(image, (nY, nX), corners, True)object_points.append(coords) # Save 3d points image_points.append(corners) # Save corresponding 2d points
Let's see the resulting image:

Figure 1.21 – Corners of the calibration image found by OpenCV
Calibration
After finding the corners in several images, we are finally ready to generate the calibration data using calibrateCamera()
:
ret, mtx, dist, rvecs, tvecs = cv2.calibrateCamera(object_points, image_points, shape[::-1], None, None)
Now, we are ready to correct our images, using undistort()
:
dst = cv2.undistort(image, mtx, dist, None, mtx)
Let's see the result:
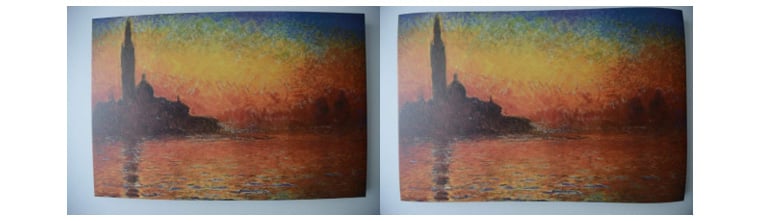
Figure 1.22 – Original image and calibrated image
We can see that the second image has less barrel distortion, but it is not great. We probably need more and better calibration samples.
But we can also try to get more precision from the same calibration images by looking for sub-pixel precision when looking for the corners. This can be done by calling cornerSubPix()
after findChessboardCorners()
:
corners = cv2.cornerSubPix(image, corners, (11, 11), (-1, -1), (cv2.TERM_CRITERIA_EPS + cv2.TERM_CRITERIA_MAX_ITER, 30, 0.001))
The following is the resulting image:
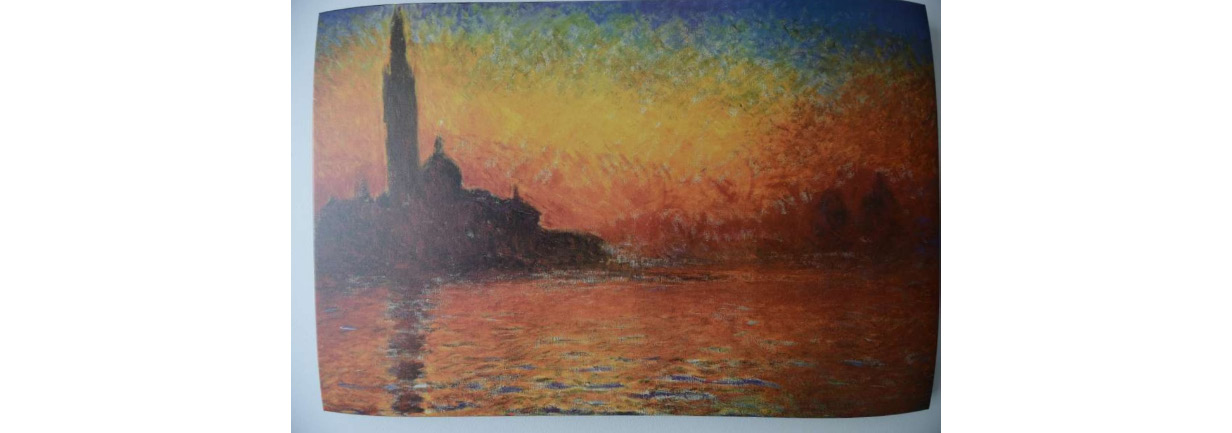
Figure 1.23 – Image calibrated with sub-pixel precision
As the complete code is a bit long, I recommend checking out the full source code on GitHub.