In this section, we are going to analyze two neural models (Sanger's and Rubner-Tavan's networks) that can perform principal component analysis (PCA) without the need of either eigendecomposing the covariance matrix or performing truncated SVD. They are both based on the concept of Hebbian learning (for further details, please refer to Dayan, P. and Abbott, L. F., Theoretical Neuroscience, The MIT Press, 2005 or Bonaccorso, G., Mastering Machine Learning Algorithms, Packt, 2018), which is one of the first mathematical theories about the dynamics of very simple neurons. Nevertheless, such concepts have very interesting implications, in particular in the field of component analysis. In order to better understand the dynamics of networks, it will be helpful to provide a quick overview of the basic model of a neuron. Let's...




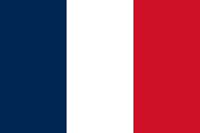


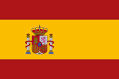



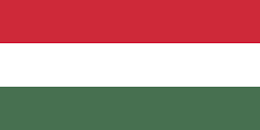

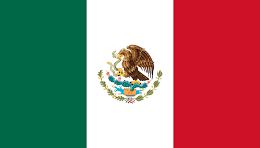


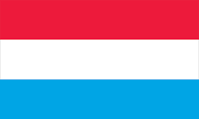



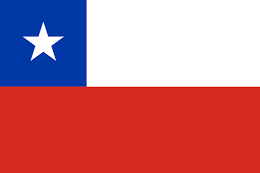


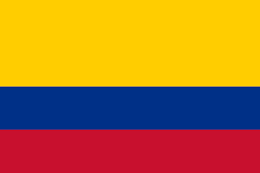
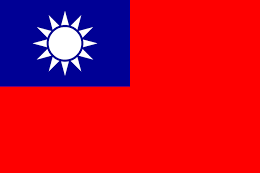


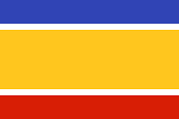
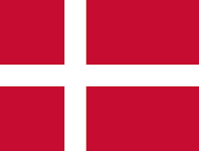
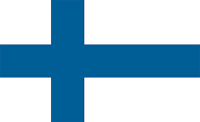



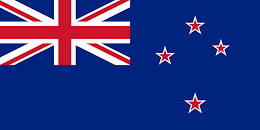





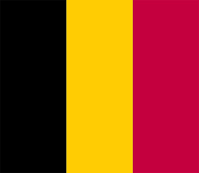

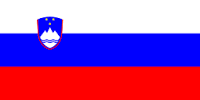




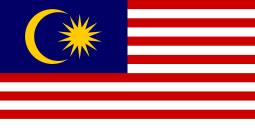


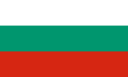


