In this chapter, we introduced the concept of GANs, discussing an example of a DCGAN. Such models have the ability to learn a data generating process through the use of two neural networks involved in a minimax game. The generator has to learn how to return samples that are indistinguishable from the others employed during the training process. The discriminator, or critic, has to become smarter and smarter in only assigning high probabilities to valid samples. The adversarial training approach is based on the idea of forcing the generator to win against the discriminator, by learning how to cheat it with synthetic samples with the same properties as the real ones. At the same time, the generator is forced to win against the discriminator by becoming more and more selective. In our examples, we also analyzed an important variant, called WGAN, which can be employed when...




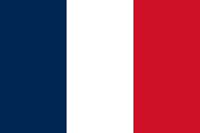


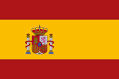



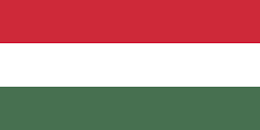

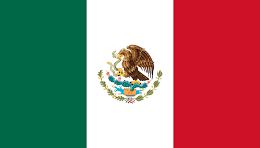


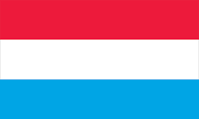



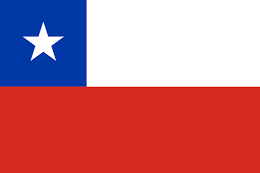


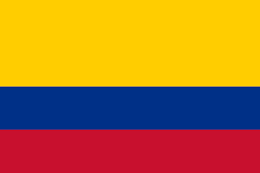
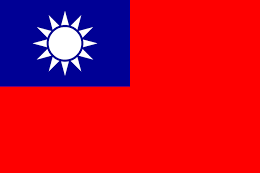


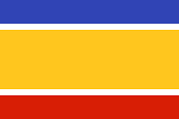
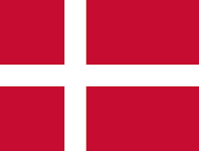
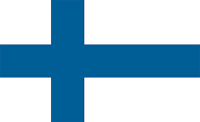



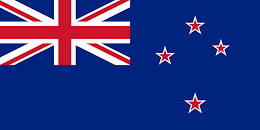





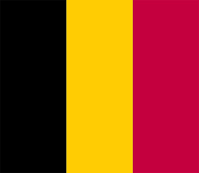

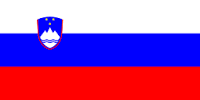




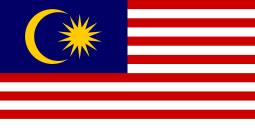


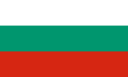


