Before we delve into these models and gain familiarity with some of these algorithms, we must learn about preprocessing the training data. We covered some of the preprocessing steps when working with text data such as tokenization, stop word removal, lemmatization, stemming, and so on in Chapter 3, Building Your NLP Vocabulary. However, there are some additional data preprocessing steps that are extremely crucial in ML as the training data needs to adhere to certain rules to be of any value to the model. Poorly processed data is guaranteed to train low accuracy models. It should be noted that data preprocessing is a vast field and that you may be required to perform various preprocessing steps based on the data you are working with. For example, you may be required to handle unstructured data; perform outlier analysis, invalid data analysis, and duplicate data analysis; identify correlated features; and more. However, we will focus on some of the most widely used preprocessing...




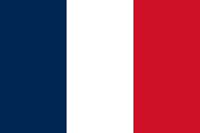


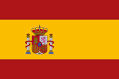



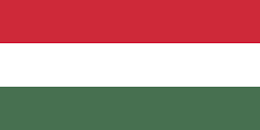

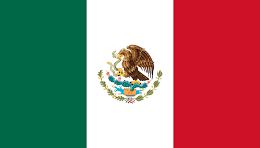


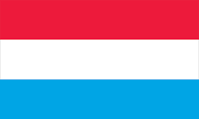



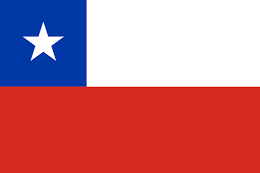


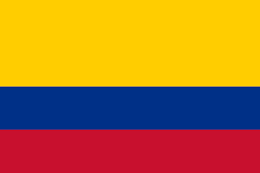
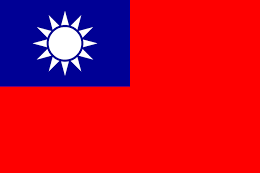


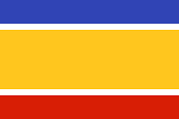
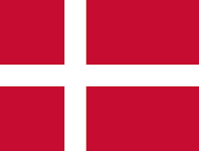
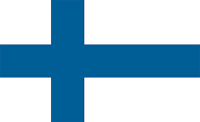



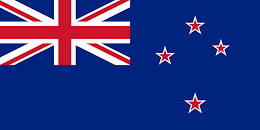





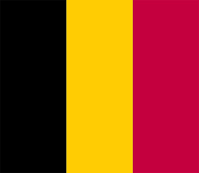

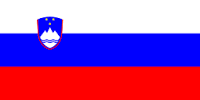




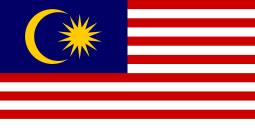


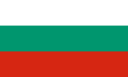


