A Siamese network, as the name suggests, is an architecture with two parallel layers. In this architecture, instead of a model learning to classify its inputs using classification loss functions, the model learns to differentiate between two given inputs. It compares two inputs based on a similarity metric and checks whether they are the same. Similar to any deep learning architecture, a Siamese network also has two phases—a training and a testing phase. But, for a one-shot learning approach (as we won't have a lot of data points), we will be training the model architecture on one dataset and testing it on a different dataset. To put this in simpler terms, we learn image embeddings using a supervised metric-based approach with Siamese neural networks, and then reuse that network's features for one-shot learning without fine-tuning...




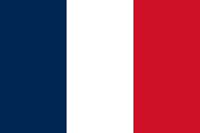


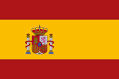



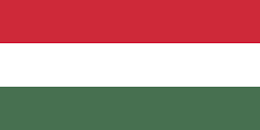

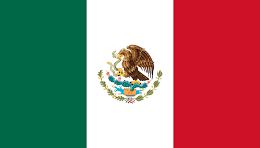


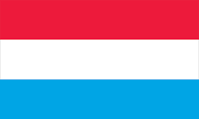



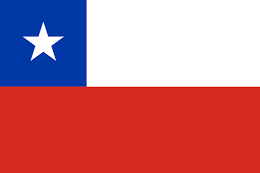


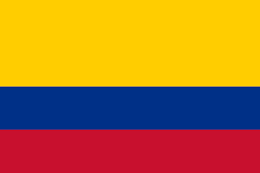
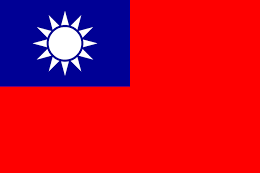


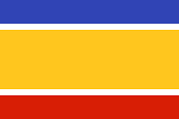
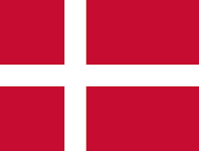
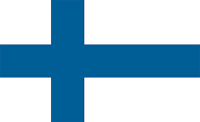



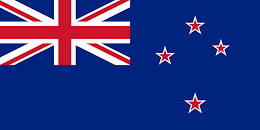





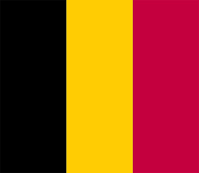

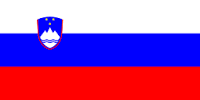




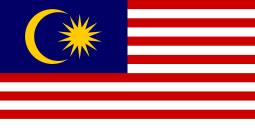


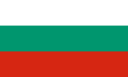


