In this section of the chapter, we implemented a specific type of GAN (that is, the DCGAN) for a specific use case (image generation). The idea of using two networks in parallel to keep each other in check, however, can be applied to various types of networks, for very different use cases. For example, if you wish to generate synthetic timeseries data, we can implement the same concepts we learned here with recurrent neural networks to design a generative adversarial model! There have been several attempts at this in the research community, with quite successful results. A group of Swedish researchers, for example, used recurrent neural networks in a generative adversarial setup to produce synthetic segments of classical music! Other prominent ideas with GANs involve using attention models (a topic unfortunately not covered by this book) to orient network perception...




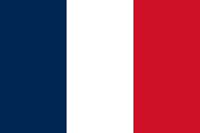


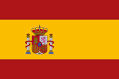



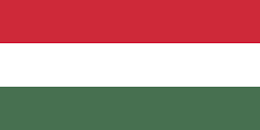

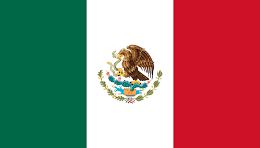


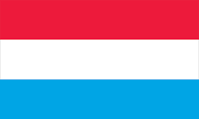



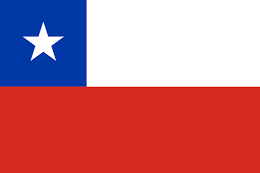


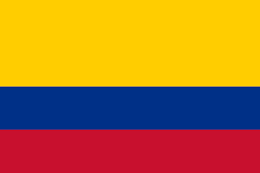
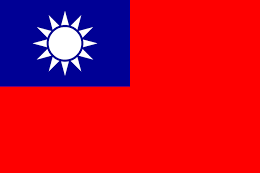


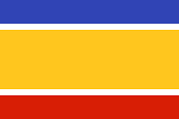
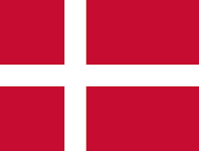
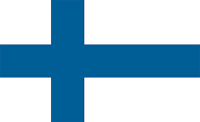



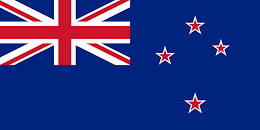





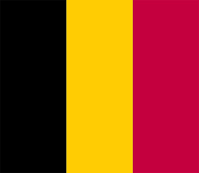

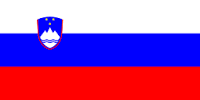




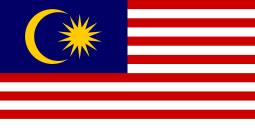


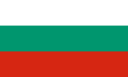


