In the preceding chapter, we familiarized ourselves with a novel area in machine learning (ML): the realm of reinforcement learning. We saw how reinforcement learning algorithms can be augmented using neural networks, and how we can learn approximate functions that can map game states to possible actions the agent may take. These actions are then compared to a moving target variable, which in turn was defined by what we called the Bellman equation. This, strictly speaking, is a self-supervised ML technique, as it is the Bellman equation that's used to compare our predictions, and not a set of labeled target variables, as would be the case for a supervised learning approach (for example, game screens labeled with optimal actions to take at each state). The latter, while possible, proves to be much more computationally intensive for the given use case. Now we will...




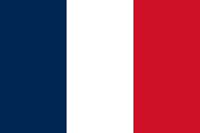


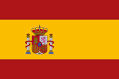



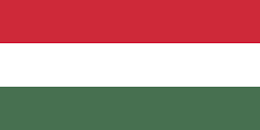

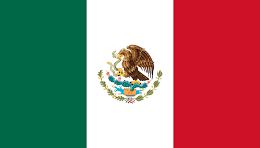


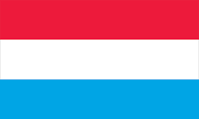



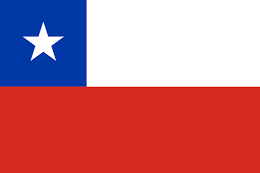


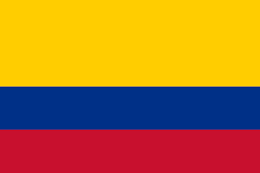
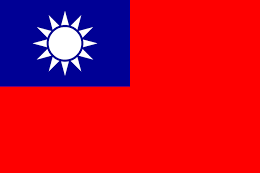


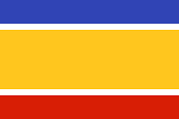
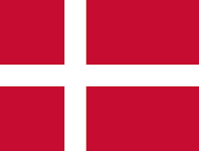
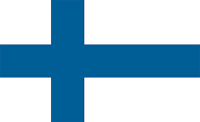



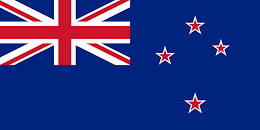





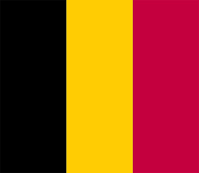

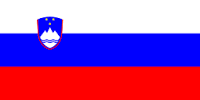




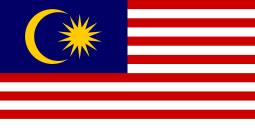


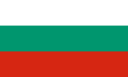


