The main goal of dimension reduction methods is to make the dimension of the transformed representation correspond with the internal dimension of the data. In other words, it should be similar to the minimum number of variables necessary to express all the possible properties of the data. Reducing the dimension helps mitigate the impact of the curse of dimensionality and other undesirable properties that occur in high-dimensional spaces. As a result, reducing dimensionality can effectively solve problems regarding classification, visualization, and compressing high-dimensional data. It makes sense to apply dimensionality reduction only when particular data is redundant; otherwise, we can lose important information. In other words, if we are able to solve the problem using data of smaller dimensions with the same level of efficiency and...




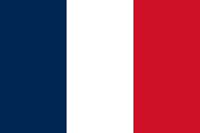


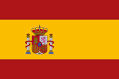



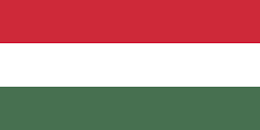

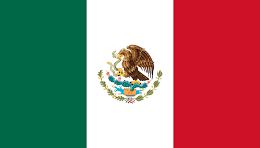


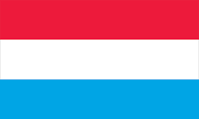



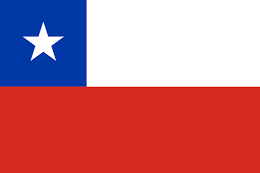


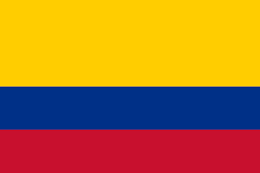
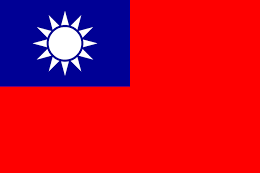


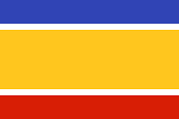
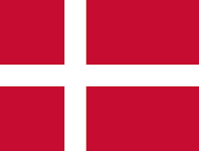
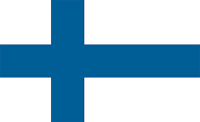



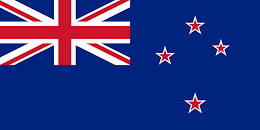





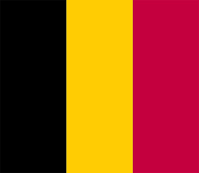

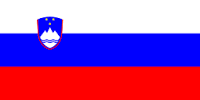




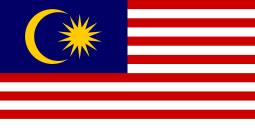


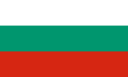


