In this chapter, we got hands-on with an actor-critic architecture-based deep reinforcement learning agent, starting from the basics. We started with the introduction to policy gradient-based methods and walked through the step-by-step process of representing the objective function for the policy gradient optimization, understanding the likelihood ratio trick, and finally deriving the policy gradient theorem. We then looked at how the actor-critic architecture makes use of the policy gradient theorem and uses an actor component to represent the policy of the agent, and a critic component to represent the state/action/advantage value function, depending on the implementation of the architecture. With an intuitive understanding of the actor-critic architecture, we moved on to the A2C algorithm and discussed the six steps involved in it. We then discussed the n-step return...




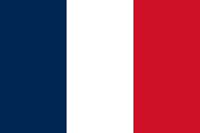


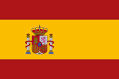



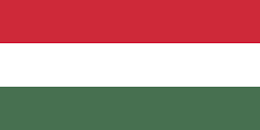

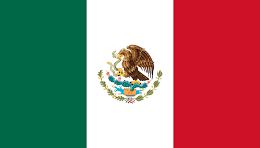


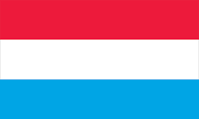



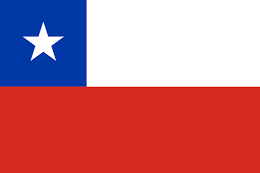


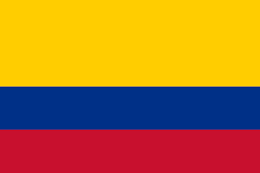
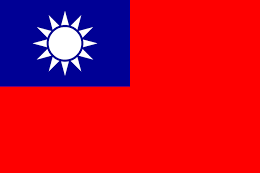


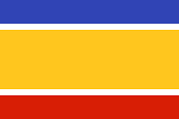
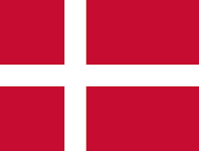
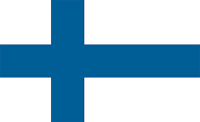



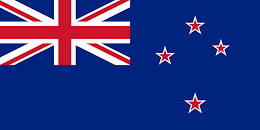





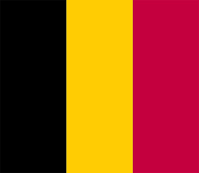

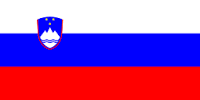




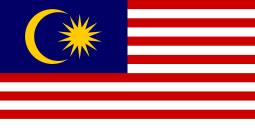


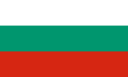


