We started this chapter with the grand goal of developing intelligent learning agents that can achieve great scores in Atari games. We made incremental progress towards it by implementing several techniques to improve upon the Q-learner that we developed in the previous chapter. We first started with learning how we can use a neural network to approximate the Q action-value function and made our learning concrete by practically implementing a shallow neural network to solve the famous Cart Pole problem. We then implemented experience memory and experience replay that enables the agent to learn from (mini) randomly sampled batches of experiences that helped in improving the performance by breaking the correlations between the agent's interactions and increasing the sample efficiency with the batch replay of the agent's prior experience. We then revisited the epsilon...




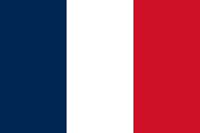


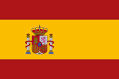



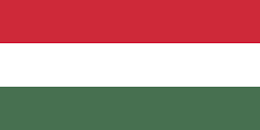

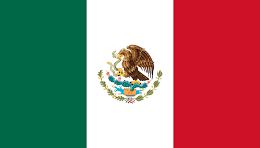


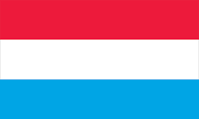



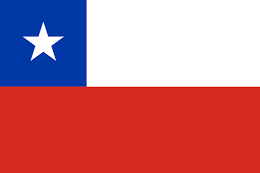


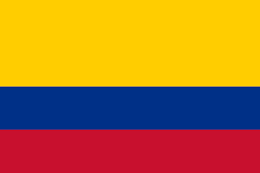
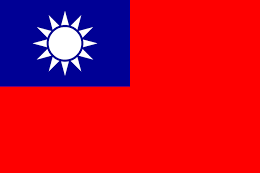


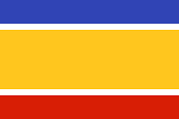
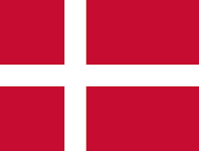
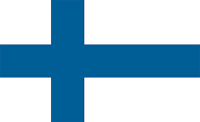



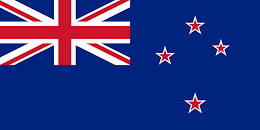





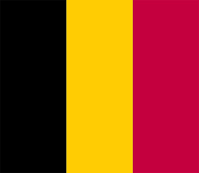

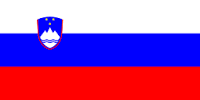




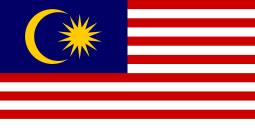


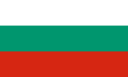


