XGBoost is a boosting library with parallel, GPU, and distributed execution support. It has helped many machine learning engineers and data scientists to win Kaggle.com competitions. Furthermore, it provides an interface that resembles scikit-learn's interface. Thus, someone already familiar with the interface is able to quickly utilize the library. Additionally, it allows for very fine control over the ensemble's creation. It supports monotonic constraints (that is, the predicted value should only increase or decrease, relative to a specific feature), as well as feature interaction constraints (for example, if a decision tree creates a node that splits by age, it should not use sex as a splitting feature for all children of that specific node). Finally, it adds an additional regularization parameter, gamma, which further reduces the overfitting capabilities...




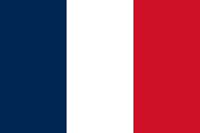


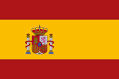



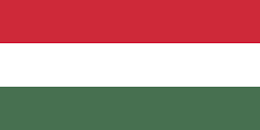

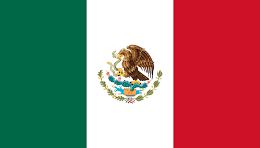


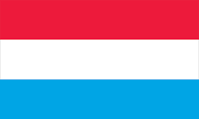



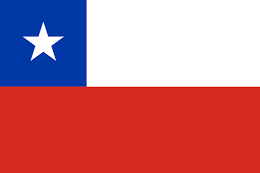


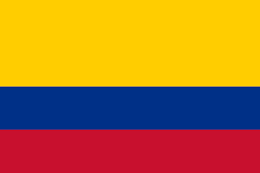
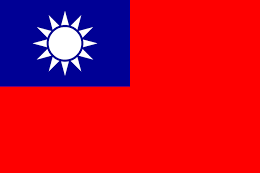


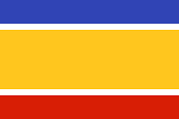
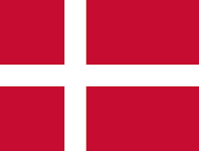
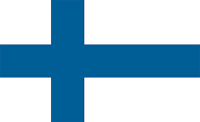



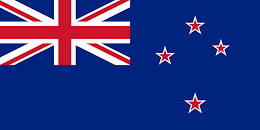





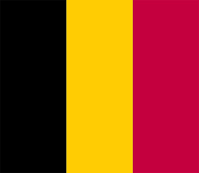

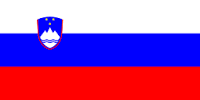




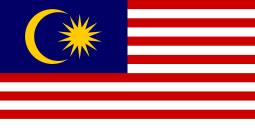


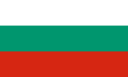


