Before any training can begin, ML techniques in general, and so DL techniques, have a set of parameters that have to be chosen. They are referred to as hyperparameters. Keeping focus on DL, we can say that some of these (the number of layers and their size) define the architecture of a neural network, while others define the learning process (learning rate, regularization, and so on). Hyperparameter optimization is an attempt to automate this process (that has a significant impact on the results achieved by training a neural network) using a dedicated software that applies some search strategies. DL4J provides a tool, Arbiter, for hyperparameter optimization of neural nets. This tool doesn't fully automate the process—a manual intervention from data scientists or developers is needed in order to specify the search spaces (the ranges of valid...




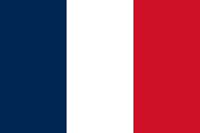


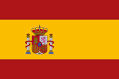



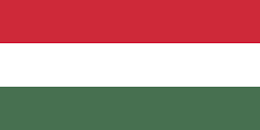

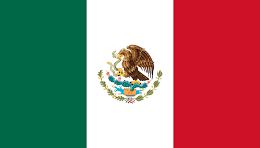


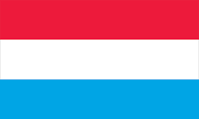



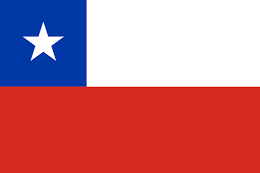


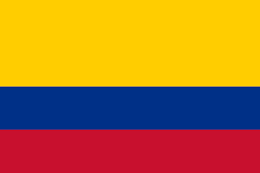
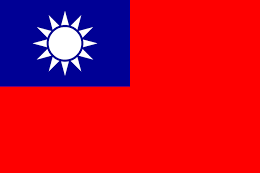


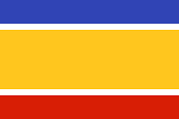
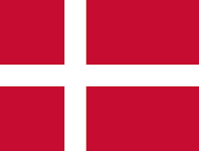
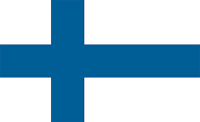



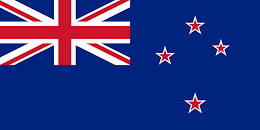





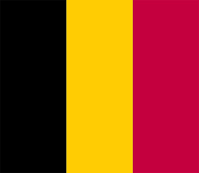

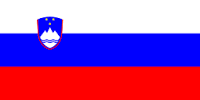




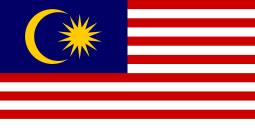


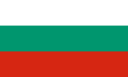


