Playing with and exploring experimental reinforcement learning environments is all well and good, but, at the end of the day, most game developers want to develop their own learning environment. To do that, we need to understand a lot more about training deep reinforcement learning environments, and, in particular, how an agent receives and processes input. Therefore, in this chapter, we will take a very close look at training one of the more difficult sample environments in Unity. This will help us understand many of the intricate details of how important input and state is to training an agent, and the many features in the Unity ML-Agents toolkit that make it easy for us to explore multiple options. This will be a critical chapter for anyone wanting to build their own environments and use the ML-Agents in their game. So, if you need to work through...




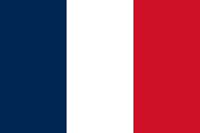


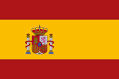



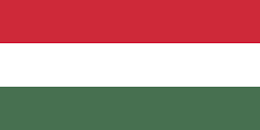

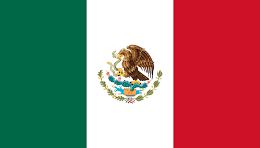


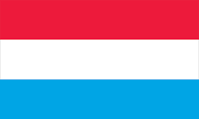



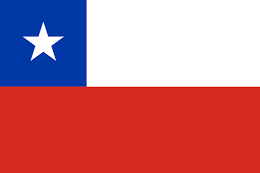


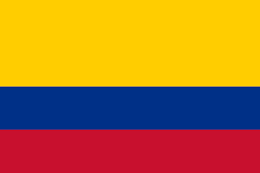
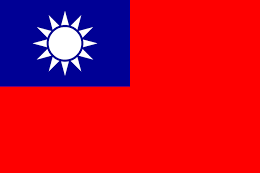


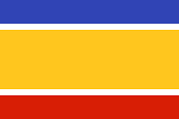
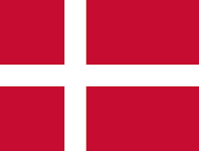
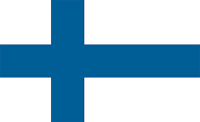



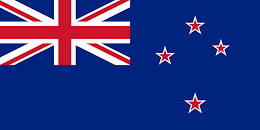





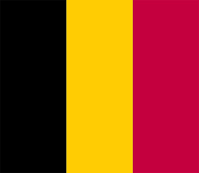

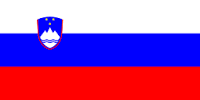




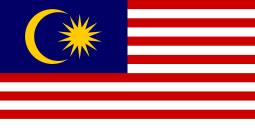


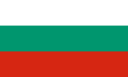


