Docker: A lightweight virtualization solution
A consistent challenge in developing robust software applications is to make them run the same on a machine different than the one on which they are developed. These differences in environments could encompass a number of variables: operating systems, programming language library versions, and hardware such as CPU models.
Traditionally, one approach to dealing with this heterogeneity has been to use a Virtual Machine (VM). While VMs are useful to run applications on diverse hardware and operating systems, they are also limited by being resource-intensive (Figure 2.3): each VM running on a host requires the overhead resources to run a completely separate operating system, along with all the applications or dependencies within the guest system.
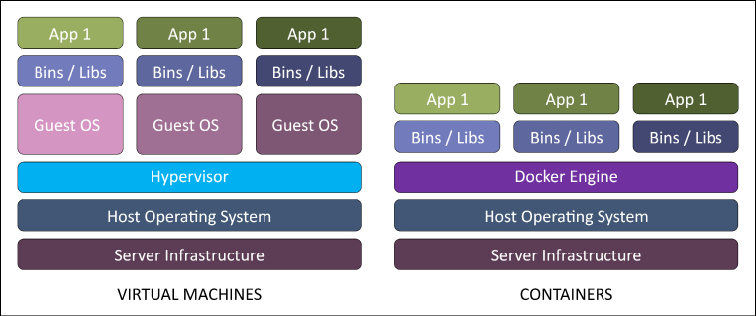
Figure 2.3: Virtual machines versus containers16
However, in some cases this is an unnecessary level of overhead; we do not necessarily need to run an entirely separate operating system, rather than just a consistent environment, including libraries and dependencies within a single operating system. This need for a lightweight framework to specify runtime environments prompted the creation of the Docker project for containerization in 2013. In essence, a container is an environment for running an application, including all dependencies and libraries, allowing reproducible deployment of web applications and other programs, such as a database or the computations in a machine learning pipeline. For our use case, we will use it to provide a reproducible Python execution environment (Python language version and libraries) to run the steps in our generative machine learning pipelines.
We will need to have Docker installed for many of the examples that will appear in the rest of this chapter and the projects in this book. For instructions on how to install Docker for your particular operating system, please refer to the directions at (https://docs.docker.com/install/). To verify that you have installed the application successfully, you should be able to run the following command on your terminal, which will print the available options:
docker run hello-world
Important Docker commands and syntax
To understand how Docker works, it is useful to walk through the template used for all Docker containers, a Dockerfile. As an example, we will use the TensorFlow container notebook example from the Kubeflow project (https://github.com/kubeflow/kubeflow/blob/master/components/example-notebook-servers/jupyter-tensorflow-full/cpu.Dockerfile).
This file is a set of instructions for how Docker should take a base operating environment, add dependencies, and execute a piece of software once it is packaged:
FROM public.ecr.aws/j1r0q0g6/notebooks/notebook-servers/jupyter-tensorflow:master-abf9ec48
# install - requirements.txt
COPY --chown=jovyan:users requirements.txt /tmp/requirements.txt
RUN python3 -m pip install -r /tmp/requirements.txt --quiet --no-cache-dir \
&& rm -f /tmp/requirements.txt
While the exact commands will differ between containers, this will give you a flavor for the way we can use containers to manage an application – in this case running a Jupyter notebook for interactive machine learning experimentation using a consistent set of libraries. Once we have installed the Docker runtime for our particular operating system, we would execute such a file by running:
Docker build -f <Dockerfilename> -t <image name:tag>
When we do this, a number of things happen. First, we retrieve the base
filesystem, or image
, from a remote repository, which is not unlike the way we collect JAR files from Artifactory when using Java build tools such as Gradle or Maven, or Python's pip installer. With this filesystem or image
, we then set required variables for the Docker build
command such as the username and TensorFlow version, and runtime environment variables for the container. We determine what shell program will be used to run the command, then we install dependencies we will need to run TensorFlow and the notebook application, and we specify the command that is run when the Docker container is started. Then we save this snapshot with an identifier composed of a base image name
and one or more tags
(such as version numbers, or, in many cases, simply a timestamp to uniquely identify this image). Finally, to actually start the notebook server running this container, we would issue the command:
Docker run <image name:tag>
By default, Docker will run the executable command in the Dockerfile
file; in our present example, that is the command to start the notebook server. However, this does not have to be the case; we could have a Dockerfile
that simply builds an execution environment for an application, and issue a command to run within that environment. In that case, the command would look like:
Docker run <image name:tag> <command>
The Docker
run commands allow us to test that our application can successfully run within the environment specified by the Dockerfile
; however, we usually want to run this application in the cloud where we can take advantage of distributed computing resources or the ability to host web applications exposed to the world at large, not locally. To do so, we need to move our image we have built to a remote repository, which may or may not be the same one we pulled the initial image from, using the push command:
Docker push <image name:tag>
Note that the image name can contain a reference to a particular registry, such as a local registry or one hosted on one of the major cloud providers such as Elastic Container Service (ECS) on AWS, Azure Kubernetes Service (AKS), or Google Container Registry. Publishing to a remote registry allows developers to share images, and us to make containers accessible to deploy in the cloud.
Connecting Docker containers with docker-compose
So far we have only discussed a few basic Docker commands, which would allow us to run a single service in a single container. However, you can probably appreciate that in the "real world" we usually need to have one or more applications running concurrently – for example, a website will have both a web application that fetches and processes data in response to activity from an end user and a database instance to log that information. In complex applications, the website might even be composed of multiple small web applications or microservices that are specialized to particular use cases such as the front end, user data, or an order management system. For these kinds of applications, we will need to have more than one container communicating with each other. The docker-compose tool (https://docs.docker.com/compose/) is written with such applications in mind: it allows us to specify several Docker containers in an application file using the YAML
format. For example, a configuration for a website with an instance of the Redis database might look like:
version: '3'
services:
web:
build: .
ports:
- "5000:5000"
volumes:
- .:/code
- logvolume01:/var/log
links:
- redis
redis:
image: redis
volumes:
logvolume01: {}
Code 2.1: A yaml input file for Docker Compose
The two application containers here are web
and the redis
database. The file also specified the volumes (disks) linked to these two applications. Using this configuration, we can run the command:
docker-compose up
This starts all the containers specified in the YAML file and allows them to communicate with each other. However, even though Docker containers and docker-compose allow us to construct complex applications using consistent execution environments, we may potentially run into issues with robustness when we deploy these services to the cloud. For example, in a web application, we cannot be assured that the virtual machines that the application is running on will persist over long periods of time, so we need processes to manage self-healing and redundancy. This is also relevant to distributed machine learning pipelines, in which we do not want to have to kill an entire job because one node in a cluster goes down, which requires us to have backup logic to restart a sub-segment of work. Also, while Docker has the docker-compose functionality to link together several containers in an application, it does not have robust rules for how communication should happen among those containers, or how to manage them as a unit. For these purposes, we turn to the Kubernetes library.