Similarity learning is the process of training a metric to compute the similarity between two entities. This could also be termed as metric learning, as the similarity is learned. A metric could be Euclidean or cosine or some other custom distance function. Entities could be any data such as an image, video, text or tables. To compute a metric, a vector representation of the image is required. This representation can be the features computed by a CNN as described in Chapter 3, Image Retrieval. The CNN that was learned for object classification can be used as the vector to compute the metric. The feature vector obtained for image classification would not be the best representation of the task at hand. In similarity learning, we find out about CNNs that generate features trained for a similarity learning task. Some applications of similarity learning...




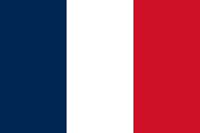


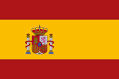



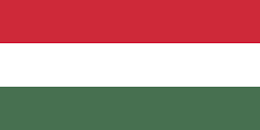

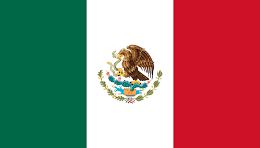


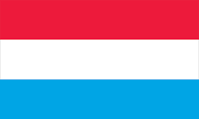



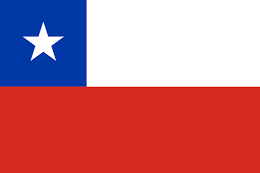


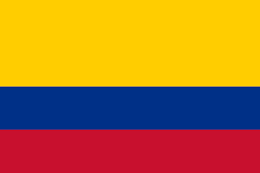
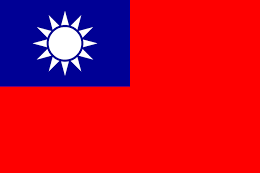


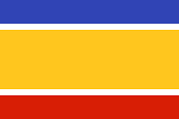
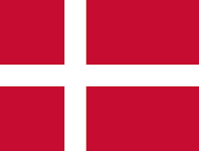
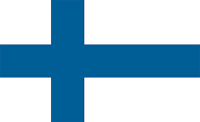



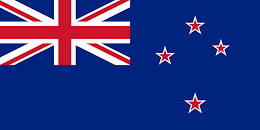





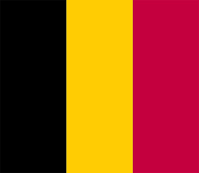

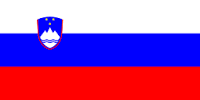




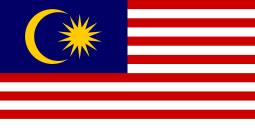


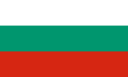


