Now we have a general understanding of deep learning and its technical advantages over traditional methods. But how do we benefit from it in reality? In this section, we will introduce how deep learning makes tremendous impact in some practical applications across a variety of fields.
Applications
Lucrative applications
In the past few years, the number of researchers and engineers in deep learning has grown at an exponential rate. Deep learning breaks new ground in almost every domain it touches using novel neural networks architectures and advanced machine learning frameworks. With significant hardware and algorithmic developments, deep learning has revolutionized the industry and has been highly successful in tackling many real-world AI and data mining problems.
We have seen an explosion in new and lucrative applications using deep learning frameworks in areas as diverse as image recognition, image search, object detection, computer vision, optical character recognition, video parsing, face recognition, pose estimation (Cao and others, Realtime Multi-Person 2D Pose Estimation using Part Affinity Fields, 2016), speech recognition, spam detection, text to speech or image caption, translation, natural language processing, chatbots, targeted online advertising serving, click-through optimization, robotics, computer vision, energy optimization, medicine, art, music, physics, autonomous car driving, data mining of biological data, bioinformatics (protein sequence prediction, phylogenetic inferences, multiple sequence alignment) big data analytics, semantic indexing, sentiment analysis, web search/information retrieval, games (Atari (http://karpathy.github.io/2016/05/31/rl/) and AlphaGo (https://deepmind.com/research/alphago/)), and beyond.
Success stories
In this section, we will enumerate a few major application areas and their success stories.
In the area of computer vision, image recognition/object recognition refers to the task of using an image or a patch of an image as input and predicting what the image or patch contains. For example, an image can be labeled dog, cat, house, bicycle, and so on. In the past, researchers were stuck at how to design good features to tackle challenging problems such as scale-invariant, orientation invariant, and so on. Some of the well-known feature descriptors are Haar-like, Histogram of Oriented Gradient (HOG), Scale-Invariant Feature Transform (SIFT), and Speeded-Up Robust Feature (SURF). While human designed features are good at certain tasks, such as HOG for human detection, it is far from ideal.
Until 2012, deep learning stunned the field with its resounding success at the ImageNet Large Scale Visual Recognition Challenge (ILSVRC). In that competition, a convolutional neural network (often called AlexNet, see the following figure), developed by Alex Krizhevsky, Ilya Sutskever, and Geoffrey Hinton won 1st place with an astounding 85% accuracy—11% better than the algorithm that won the second place! In 2013, all winning entries were based on deep learning, and by 2015 multiple CNN-based algorithms had surpassed the human recognition rate of 95%. Details can be found at their publication Delving Deep into Rectifiers: Surpassing Human-Level Performance on ImageNet Classification:
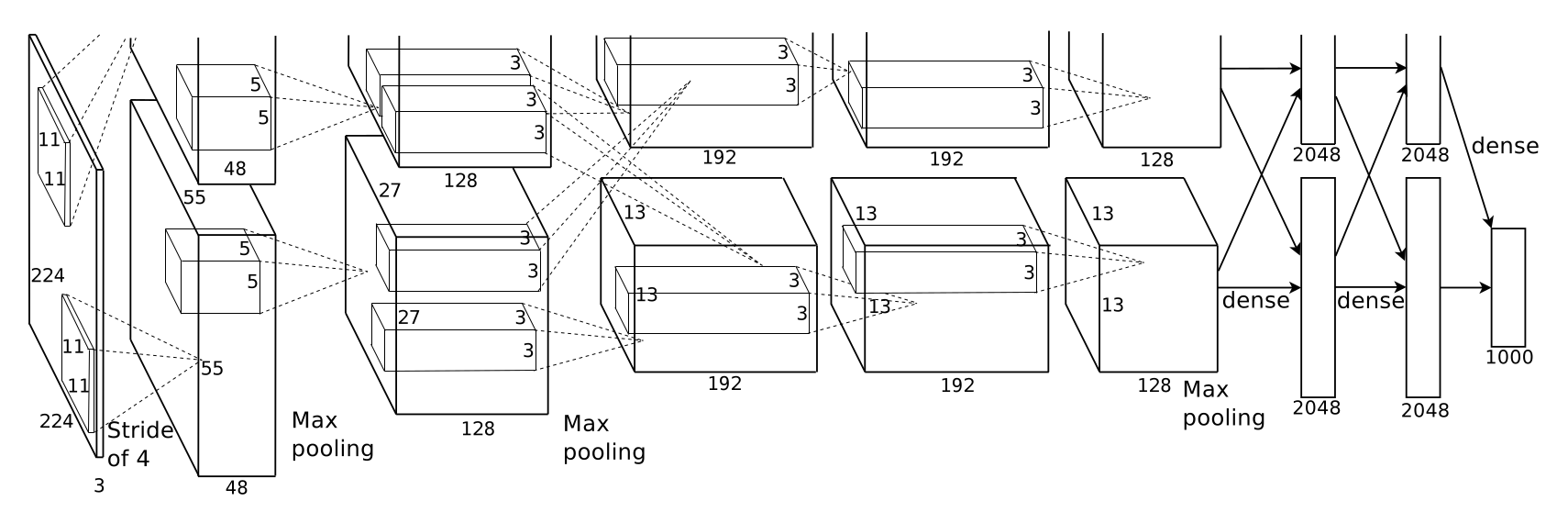
In other areas of computer vision, deep learning also shows surprising and interesting power in mimicking human intelligence. For example, deep learning cannot only identify various elements in the picture accurately (and locate them), it can also understand interesting areas such as humans and organize words/phrases into sentences to describe what’s happening in the picture. For more details, one can refer to the work presented by Andrej Karpathy and Fei-Fei Li at http://cs.stanford.edu/people/karpathy/deepimagesent/. They trained a deep learning network to identify dozens of interesting areas and objects, and described the subjects and movements in the picture with correct English grammar. This involves training on both image information and language information to make the right connection between them.
As a further progress, Justin Johnson, Andrej Karpathy and Feifei Li published a new work in 2016 called DenseCap: Fully Convolutional Localization Networks for Dense Captioning. Their proposed fully Convolutional Localization Network (FCLN) architecture can localize and describe salient regions in images in natural language. Some examples are shown in the following figure:
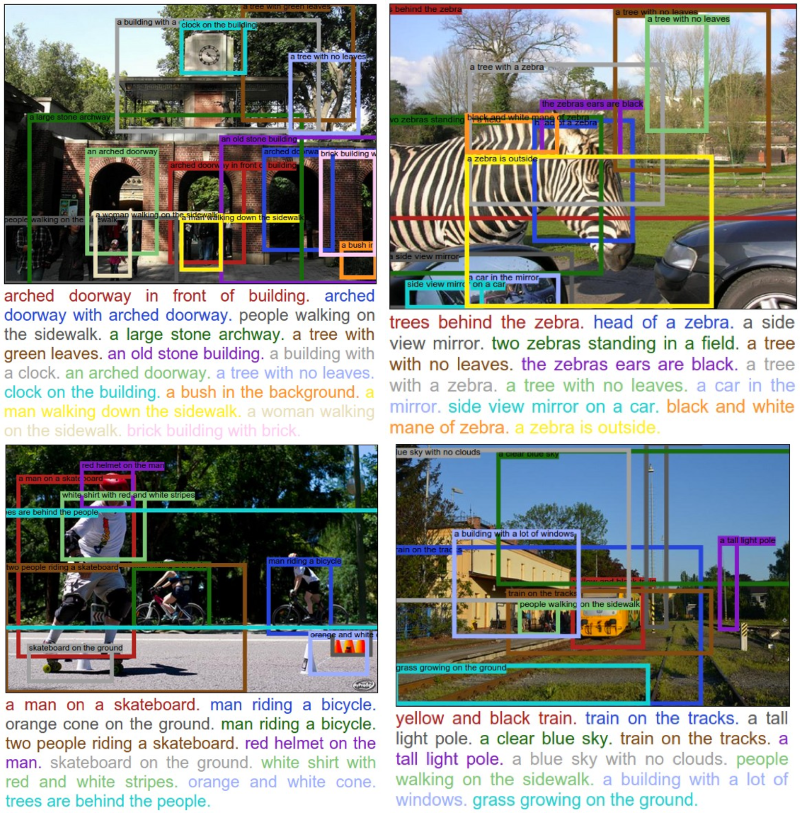
Recently, attention-based neural encoder-decoder frameworks have been widely adopted for image captioning, where novel adaptive attention models with a visual sentinel have been incorporated and better performance has been achieved. Details can be found at their work of Knowing When to Look: Adaptive Attention via A Visual Sentinel for Image Captioning.
Early in 2017, Ryan Dahl and others from the Google Brain team proposed a deep learning network called Pixel Recursive Super Resolution to take very low-resolution images of faces and enhance their resolution significantly. It can predict what each face most likely looks like. For example, in the following figure, in the left-hand column, you can see the original 8 x 8 photos, the prediction results in the middle can be found fairly close to the ground truth (in the very right column):
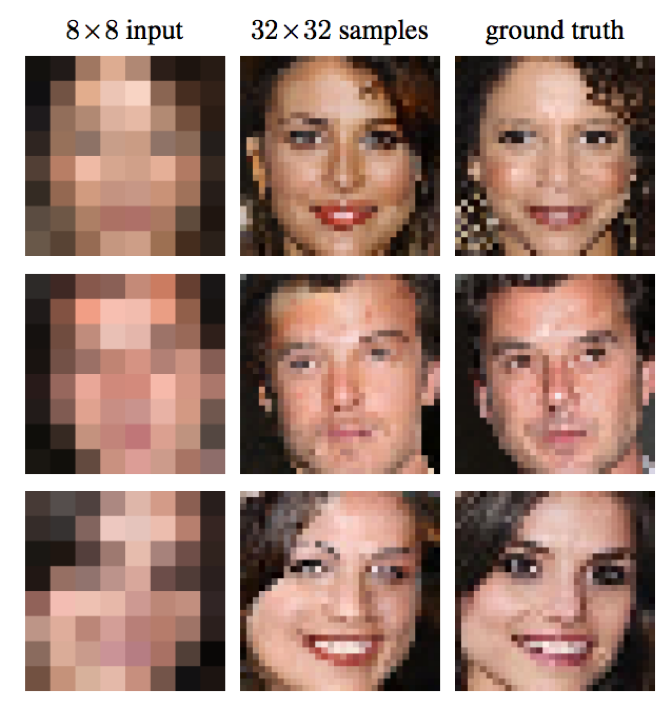
In the area of semantic indexing for search engines, given the advantages of automated feature representation by deep learning, data in various formats can now be represented in a more efficient and useful manner. This provides a powerful source of knowledge discovery and comprehension in addition to increased speed and efficiency. Microsoft Audio Video Indexing Service (MAVIS) is an example that uses deep learning (ANN)-based speech recognition to enable searching for audio and video files with speech.
In the area of natural language processing (NLP), word/character representation learning (such as Word2Vec) and machine translation are great practical examples. In fact, in the past two or three years, deep learning has almost replaced traditional machine translation.
Machine translation is automated translation, which typically refers to statistical inference-based systems that deliver more fluent-sounding but less consistent translations for speech or text between various languages. In the past, popular methods have been statistical techniques that learn the translation rules from a large corpus, as a replacement for a language expert. While cases like this overcome the bottleneck of data acquisition, many challenges exist. For example, hand-crafted features may not be ideal as they cannot cover all possible linguistic variations. It is difficult to use global features, the translation module heavily relies on pre-processing steps including word alignment, word segmentation, tokenization, rule-extraction, syntactic parsing, and so on. The recent development of deep learning provides solutions to these challenges. A machine translator that translates through one large neural network is often called Neural Machine Translation (NMT). Essentially, it’s a sequence to sequence learning problem, where the goal of the neural networks is to learn a parameterized function of P (yT | x1..N, y1..T-1) that maps from the input sequence/source sentence to the output sequence/target sentence. The mapping function often contains two stages: encoding and decoding. The encoder maps a source sequence x1..N to one or more vectors to produce hidden state representations. The decoder predicts a target sequence y1..M symbol by symbol using the source sequence vector representations and previously predicted symbols.
As illustrated by the the following figure, this vase-like shape produces good representation/embeddings at the middle hidden layer:
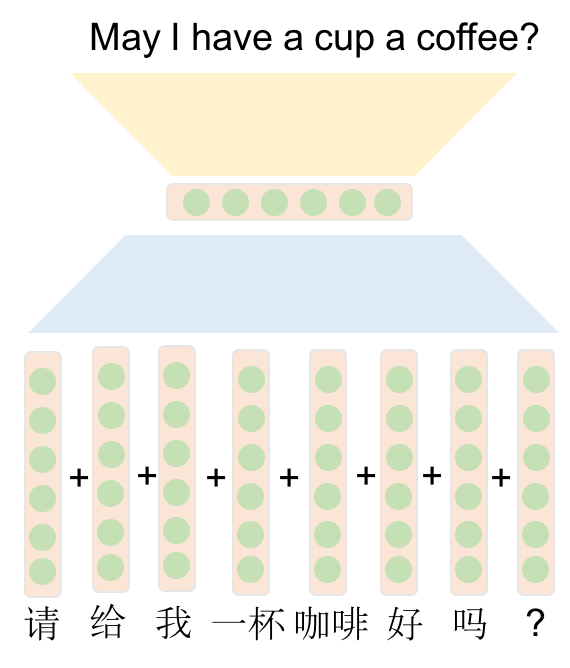
However, NMT systems are known to be computationally expensive both in training and in translation inference. Also, most NMT systems have difficulty with rare words. Some recent improvements include the attention mechanism (Bahdanau and others, Neural Machine Translation by Jointly Learning to Align and Translate, 2014), Subword level modelling (Sennrich and others, Neural Machine Translation of Rare Words with Subword Units, 2015) and character level translation, and the improvements of loss function (Chung and others, A Character-Level Decoder without Explicit Segmentation for Neural Machine Translation 2016). In 2016, Google launched their own NMT system to work on a notoriously difficult language pair, Chinese to English and tried to overcome these disadvantages.
Google’s NMT system (GNMT) conducts about 18 million translations per day from Chinese to English. The production deployment is built on top of the publicly available machine learning toolkit TensorFlow (https://www.tensorflow.org/) and Google’s Tensor Processing Units (TPUs), which provide sufficient computational power to deploy these powerful GNMT models while meeting the stringent latency requirements. The model itself is a deep LSTM model with eight encoder and eight decoder layers using attention and residual connections. On the WMT'14 English-to-French and English-to-German benchmarks, GNMT achieves competitive results. Using a human side-by-side evaluation on a set of isolated simple sentences, it reduces translation errors by an average of 60% compared to Google's phrase-based production system. For more details, one can refer to their tech blog (https://research.googleblog.com/2016/09/a-neural-network-for-machine.html) or paper (Wu and others, Google's Neural Machine Translation System: Bridging the Gap between Human and Machine Translation, 2016). The following figure shows the improvements per language pairs by the deep learning system. One can see that for French -> English, it is almost as good as a human translator:
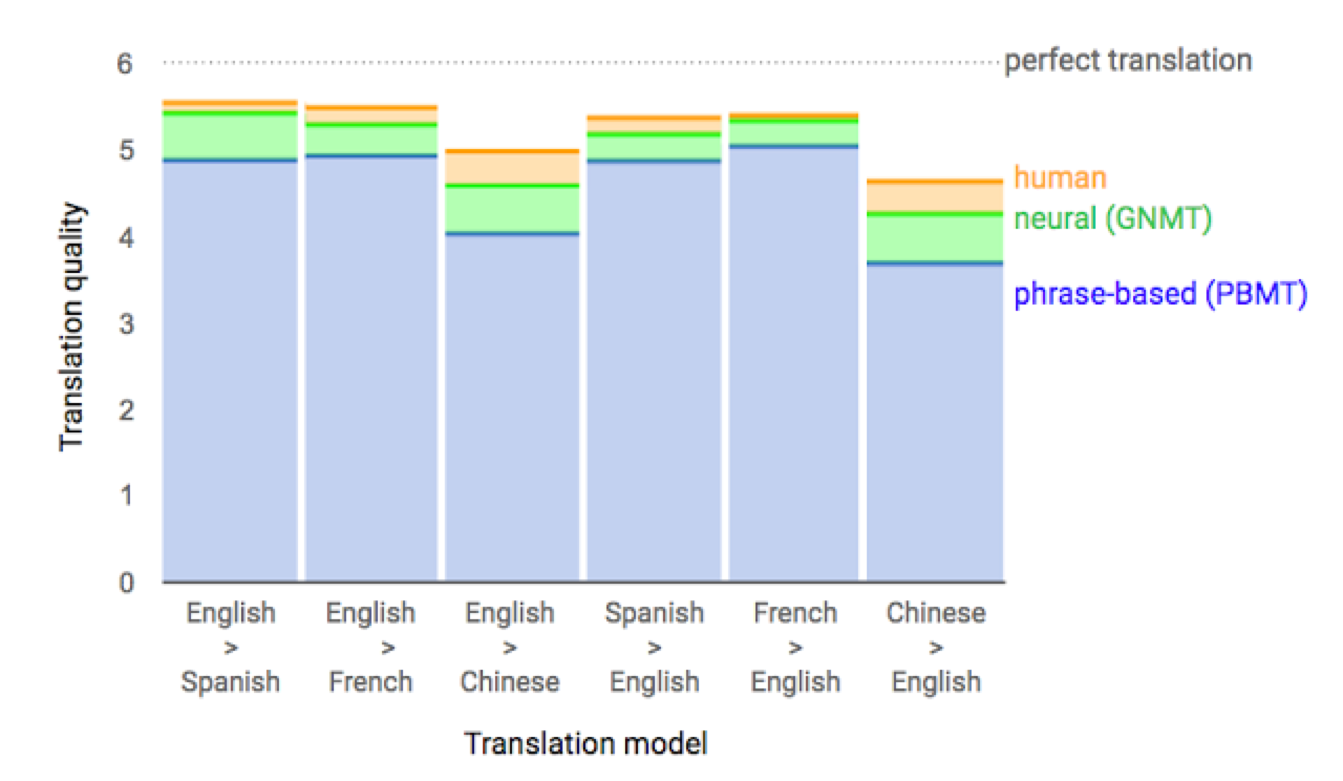
In 2016, Google released WaveNet (https://deepmind.com/blog/wavenet-generative-model-raw-audio/) and Baidu released deep speech, both are deep learning networks that generated voice automatically. The systems learn to mimic human voices by themselves and improve over time, and it is getting harder and harder for an audience to differentiate them from a real human speaking. Why is this important? Although Siri (https://www.wikiwand.com/en/Siri) and Alexa (https://www.wikiwand.com/en/Amazon_Alexa) can talk well, in the past, text2voice systems were mostly manually trained, which was not in a completely autonomous way to create new voices.
While there is still some gap before computers can speak like humans, we are definitely a step closer to realizing automatic voice generation. In addition, deep learning has shown its impressive abilities in music composition and sound generation from videos, for example Owens and their co-authors work Visually Indicated Sounds, 2015.
Deep learning has been applied extensively in self-driving cars, from perception to localization, to path planning. In perception, deep learning is often used to detect cars and pedestrians, for example using the Single Shot MultiBox Detector (Liu and others, SSD: Single Shot MultiBox Detector, 2015) or YOLO Real-Time Object Detection (Redmon and others, You Only Look Once: Unified, Real-Time Object Detection, 2015). People can also use deep learning to understand the scene the car is seeing, for example, the SegNet (Badrinarayanan, SegNet: A Deep Convolutional Encoder-Decoder Architecture for Image Segmentation, 2015), segmenting the scene into pieces with semantic meaning (sky, building, pole, road, fence, vehicle, bike, pedestrian, and so on). In localization, deep learning can be used to perform odometry, for example, VINet (Clark and others, VINet: Visual-Inertial Odometry as a Sequence-to-Sequence Learning Problem, 2017), which estimates the exact location of the car and its pose (yaw, pitch, roll). In path planning where it is often formulated as an optimization problem, deep learning, specifically reinforcement learning, can also be applied, for example, the work by Shalev-Shwartz, and its co-authors (Safe, Multi-Agent, Reinforcement Learning for Autonomous Driving, 2016). In addition to its applications in different stages of the self-driving pipeline, deep learning has also been used to perform end-to-end learning, mapping raw pixels from the camera to steering commands (Bojarski and others, End to End Learning for Self-Driving Cars, 2016).
Deep learning for business
To leverage the power of deep learning for business, the first question would be how to choose the problems to solve? In an interview with Andrew Ng, he talked about his opinion, the rule of thumb is:
If we look around, we can easily find that companies today, large or small, have already applied deep learning to production with impressive performance and speed. Think about Google, Microsoft, Facebook, Apple, Amazon, IBM, and Baidu. It turns out we are using deep learning based applications and services on a daily basis.
Nowadays, Google can caption your uploaded images with multiple tags and descriptions. Its translation system is almost as good as a human translator. Its image search engine can return related images by either image queries or language-based semantic queries. Project Sunroof (https://www.google.com/get/sunroof) has been helping homeowners explore whether they should go solar - offering solar estimates for over 43 million houses across 42 states.
Apple is working hard to invest in machine learning and computer vision technologies, including the CoreML framework on iOS, Siri, and ARKit (augmented reality platform) on iOS, and their autonomous solutions including self-driving car applications.
Facebook can now automatically tag your friends. Researchers from Microsoft have won the ImageNet competition with better performance than a human annotator and improved their speech recognition system, which has now surpassed humans.
Industry leading companies have also contributed their large-scale deep learning platforms or tools in some way. For example, TensorFlow from Google, MXNet from Amazon, PaddlePaddle from Baidu, and Torch from Facebook. Just recently, Facebook and Microsoft introduced a new open ecosystem for interchangeable AI frameworks. All these toolkits provide useful abstractions for neural networks: routines for n-dimensional arrays (Tensors), simple use of different linear algebra backends (CPU/GPU), and automatic differentiation.
With so many resources and good business models available, it can be foreseen that the process from theoretical development to practical industry realization will be shortened over time.