Model estimation
Once feature sets get finalized in our last section, what follows is to estimate the parameters of the selected models, for which we can use either MLlib or R. As before, we need to arrange distributed computing.
To simplify, we can utilize Databricks' Job feature. Specifically, within the Databricks environment, we can go to Jobs to create jobs.
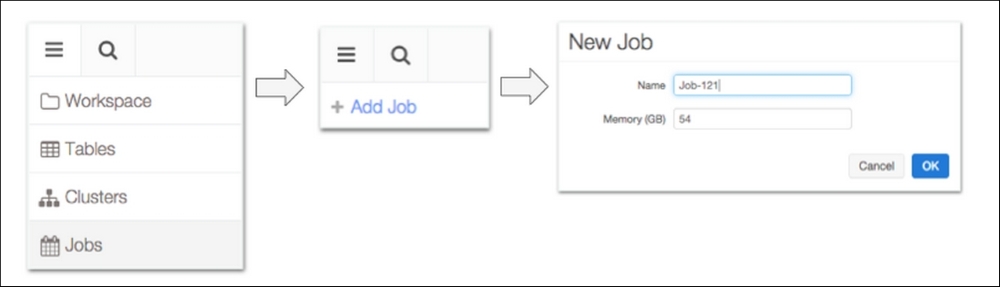
Then, users can select R notebooks to run specific clusters, and then schedule jobs. Once scheduled, users can also monitor the running and then collect the results.
In section, Methods for fraud detection, we prepared some codes for each of the three models selected. Now, we need to modify them with the final set of features, so we can create notebooks.
For now, we have one target variable prepared and 18 features, so we need to insert all of them into the code developed in section, Methods for fraud detection to finalize our notebook. Then, we will use Spark's distributed computing to get the notebook implemented in a distributed...