- Start a new project in IntelliJ or in an IDE of your choice. Make sure that the necessary JAR files are included.
- Download the sample code for the book, find the myFirstSpark20.scala file, and place the code in the following directory.
We installed Spark 2.0 in the C:\spark-2.0.0-bin-hadoop2.7\ directory on a Windows machine.
- Place the myFirstSpark20.scala file in the C:\spark-2.0.0-bin-hadoop2.7\examples\src\main\scala\spark\ml\cookbook\chapter1 directory:
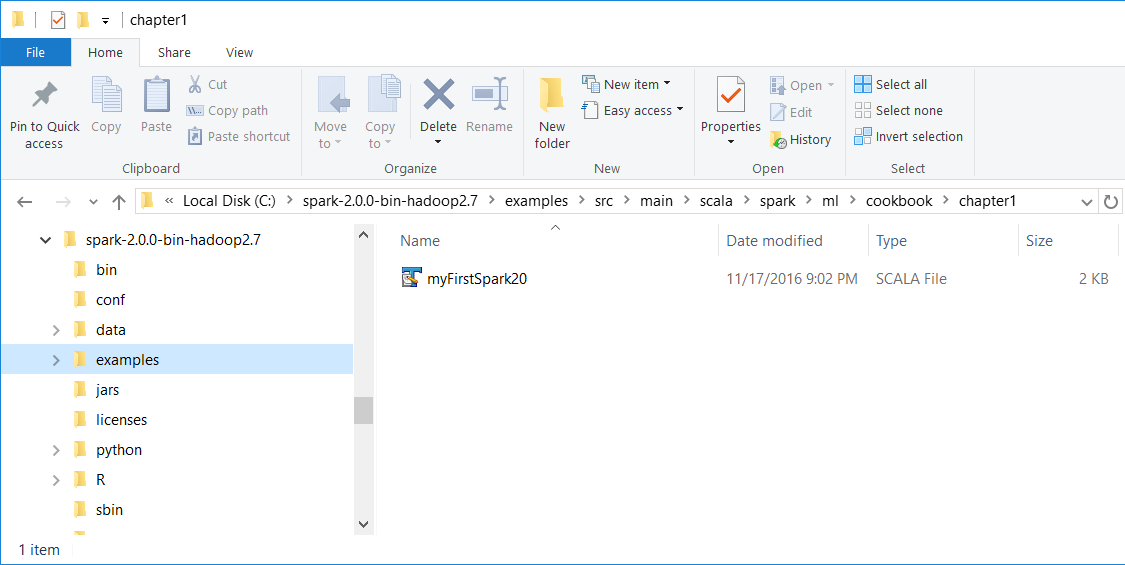
Mac users note that we installed Spark 2.0 in the /Users/USERNAME/spark/spark-2.0.0-bin-hadoop2.7/ directory on a Mac machine.
Place the myFirstSpark20.scala file in the /Users/USERNAME/spark/spark-2.0.0-bin-hadoop2.7/examples/src/main/scala/spark/ml/cookbook/chapter1 directory.
- Set up the package location where the program will reside:
package spark.ml.cookbook.chapter1
- Import the necessary packages for the Spark session to gain access to the cluster and log4j.Logger to reduce the amount of output produced by Spark:
import org.apache.spark.sql.SparkSession
import org.apache.log4j.Logger
import org.apache.log4j.Level
- Set output level to ERROR to reduce Spark's logging output:
Logger.getLogger("org").setLevel(Level.ERROR)
- Initialize a Spark session by specifying configurations with the builder pattern, thus making an entry point available for the Spark cluster:
val spark = SparkSession
.builder
.master("local[*]")
.appName("myFirstSpark20")
.config("spark.sql.warehouse.dir", ".")
.getOrCreate()
The myFirstSpark20 object will run in local mode. The previous code block is a typical way to start creating a SparkSession object.
- We then create two array variables:
val x = Array(1.0,5.0,8.0,10.0,15.0,21.0,27.0,30.0,38.0,45.0,50.0,64.0)
val y = Array(5.0,1.0,4.0,11.0,25.0,18.0,33.0,20.0,30.0,43.0,55.0,57.0)
- We then let Spark create two RDDs based on the array created before:
val xRDD = spark.sparkContext.parallelize(x)
val yRDD = spark.sparkContext.parallelize(y)
- Next, we let Spark operate on the RDD; the zip() function will create a new RDD from the two RDDs mentioned before:
val zipedRDD = xRDD.zip(yRDD)
zipedRDD.collect().foreach(println)
In the console output at runtime (more details on how to run the program in the IntelliJ IDE in the following steps), you will see this:
- Now, we sum up the value for xRDD and yRDD and calculate the new zipedRDD sum value. We also calculate the item count for zipedRDD:
val xSum = zipedRDD.map(_._1).sum()
val ySum = zipedRDD.map(_._2).sum()
val xySum= zipedRDD.map(c => c._1 * c._2).sum()
val n= zipedRDD.count()
- We print out the value calculated previously in the console:
println("RDD X Sum: " +xSum)
println("RDD Y Sum: " +ySum)
println("RDD X*Y Sum: "+xySum)
println("Total count: "+n)
Here's the console output:
- We close the program by stopping the Spark session:
spark.stop()
- Once the program is complete, the layout of myFirstSpark20.scala in the IntelliJ project explorer will look like the following:
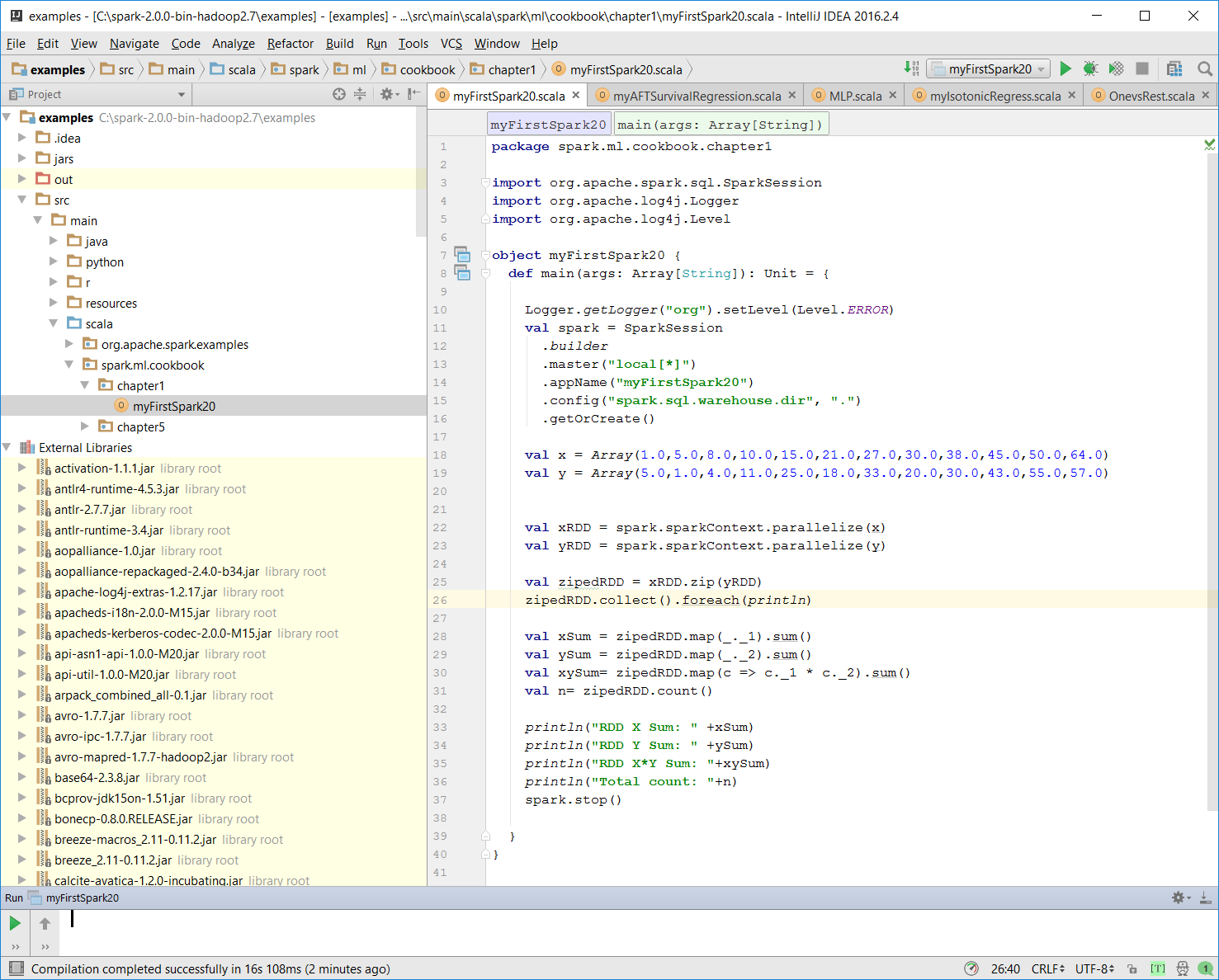
- Make sure there is no compiling error. You can test this by rebuilding the project:
Once the rebuild is complete, there should be a build completed message on the console:
Information: November 18, 2016, 11:46 AM - Compilation completed successfully with 1 warning in 55s 648ms
- You can run the previous program by right-clicking on the myFirstSpark20 object in the project explorer and selecting the context menu option (shown in the next screenshot) called Run myFirstSpark20.
You can also use the Run menu from the menu bar to perform the same action.
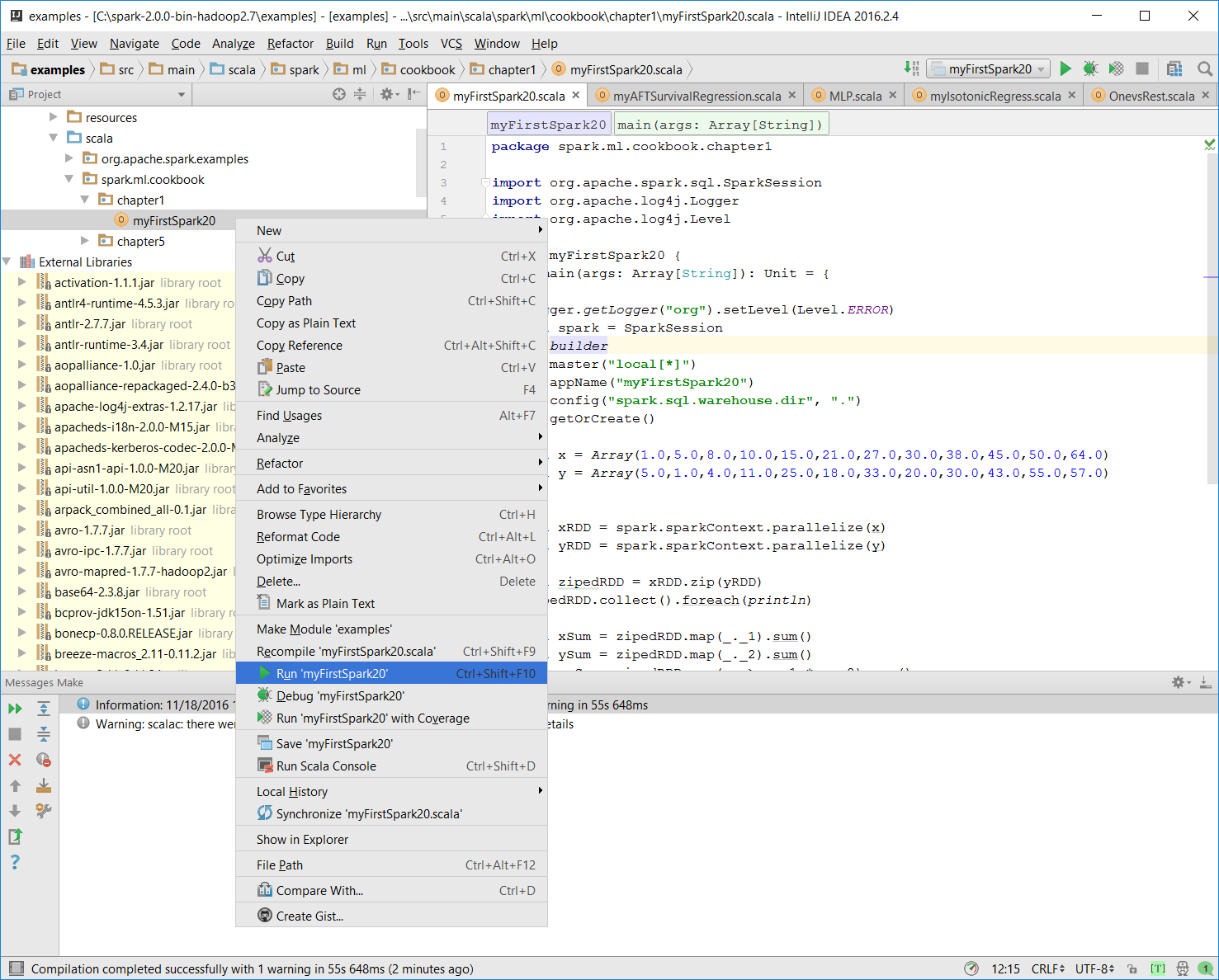
- Once the program is successfully executed, you will see the following message:
Process finished with exit code 0
This is also shown in the following screenshot:
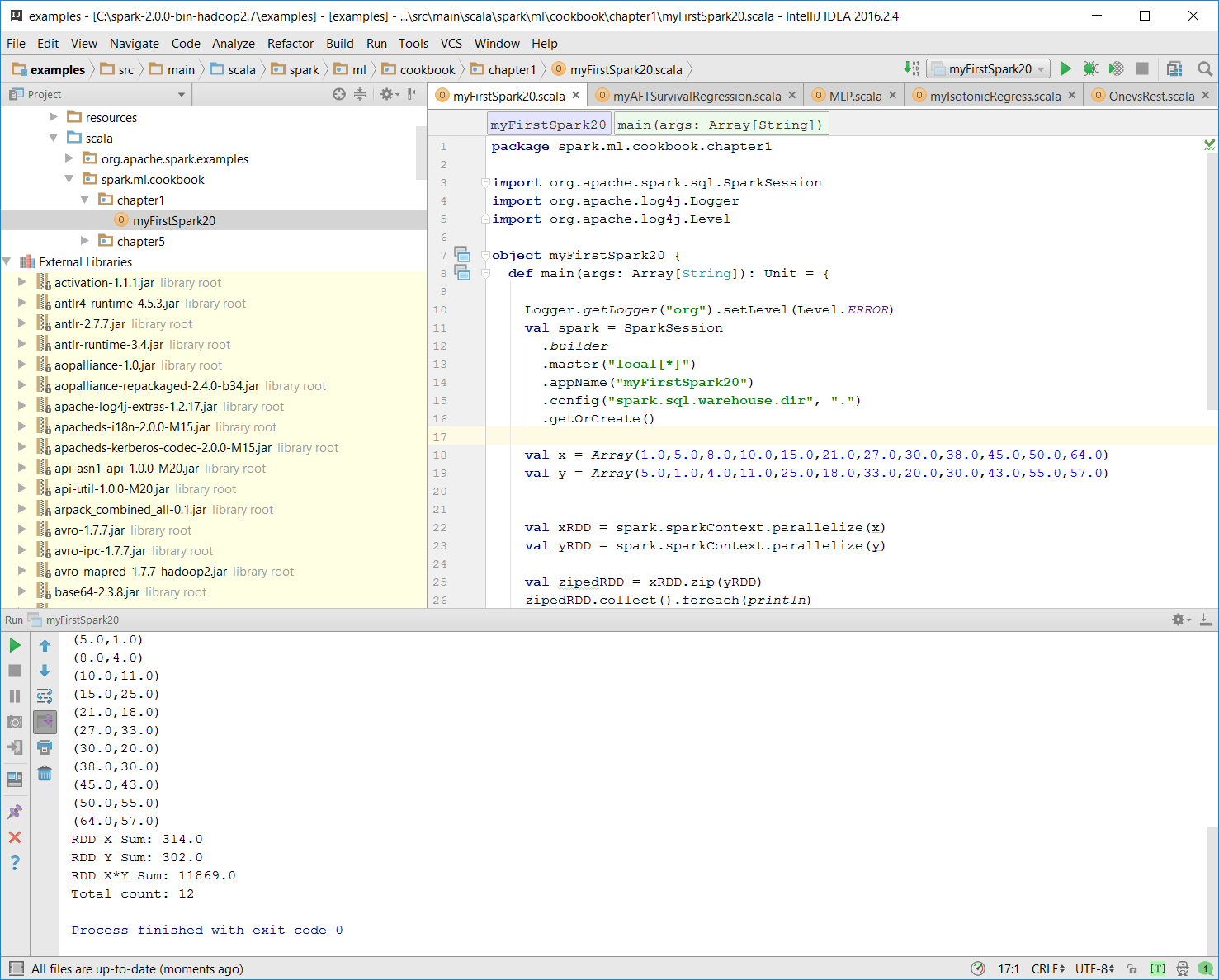
- Mac users with IntelliJ will be able to perform this action using the same context menu.
Place the code in the correct path.