- Unified DataFrame and Dataset: The Spark 2.X release has unified both the APIs. Now Dataframe is just a row in Dataset without any data type information implicitly attached.
- SparkSession: Prior to Spark 2.X, there were different entry points for different Spark jobs; that is, for Spark SQL we had sqlContext and if Hive features were also required then HiveContext was the entry point. With Spark 2.X this ambiguity has been removed and now we have one single entry point called SparkSession. However, it is to be noted that all the module-specific entry points are still very much around and have not been deprecated yet.
- Catalog API: Spark 2.X has introduced the Catalog API for accessing metadata information in Spark SQL. It can be seen as parallel to Hcatalog in Hive. It is a great step in unifying the metadata structure around Spark SQL so that the very same metadata can be exposed to non-Spark SQL applications. It is also helpful in debugging the temporary registered table in a Spark SQL session. Metadata of both sqlContext and HiveContext are available now, as the Catalog API can be accessed by SparkSession.
- Structured streaming: Structured streaming makes Spark SQL available in streaming job by continuously running the Spark SQL job and aggregating the updated results on a streaming datasets. The Dataframe and Dataset are available for operations in structured streaming along with the windowing function.
- Whole-stage code generation: The code generation engine has been modified to generate more performance-oriented code by avoiding virtual function dispatches, transfer of intermediate operation data to memory, and so on.
- Accumulator API: A new simpler and more performant Accumulator API has been added to the Spark 2.X release and the older API has been deprecated.
- A native SQL parser that supports both ANSI-SQL as well as Hive SQL has been introduced in the current Spark build.
- Hive-style bucketing support too has been added to the list of supported SQL functions in Spark SQL.
- Subquery support has been added in Spark SQL and supports other variations of the clause such as NOT IN, IN, EXISTS, and so on.
- Native CSV data source, based on the databricks implementation has been incorporated in Spark.
- The new spark.ml package which is based on Dataframe has been introduced with an objective to deprecate spark.mllib once the newly introduced package matures enough in features to replace the old package.
- Machine learning pipelines and models can now be persisted across all languages supported by Spark.




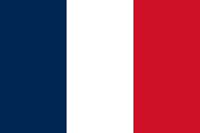


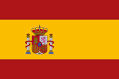



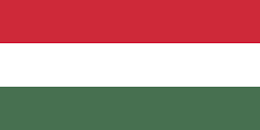

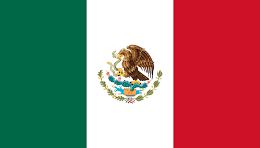


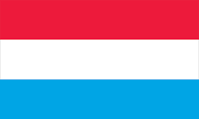



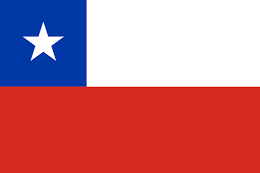


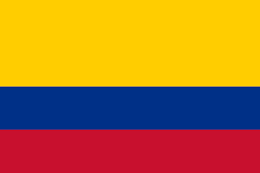
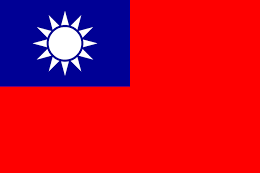


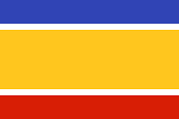
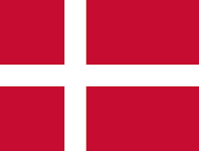
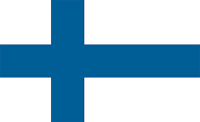



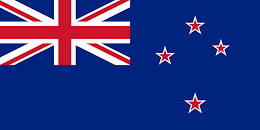





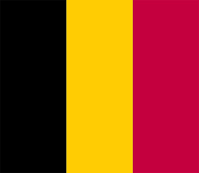

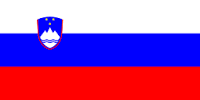




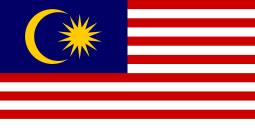


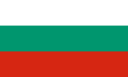


