AI solution
As always, the AI solution for deep Q-learning consists of two parts:
- Brain – the neural network that will learn and take actions
- Experience replay memory – the memory that will store our experience; the neural network will learn from this memory
Let's tackle those now!
The brain
This part of the AI solution will be responsible for teaching, storing, and evaluating our neural network. To build it, we're going to use a CNN!
Why a CNN? When explaining the theory behind them, I mentioned that they're often used when "our environment as state returns images," and that's exactly what we're dealing with here. We've already established that the game state is going to be a stacked 3D array containing the last few game frames.
In the previous chapter, we discussed that a CNN takes a 2D image as input, not a stacked 3D array of images; but do you remember this graphic?
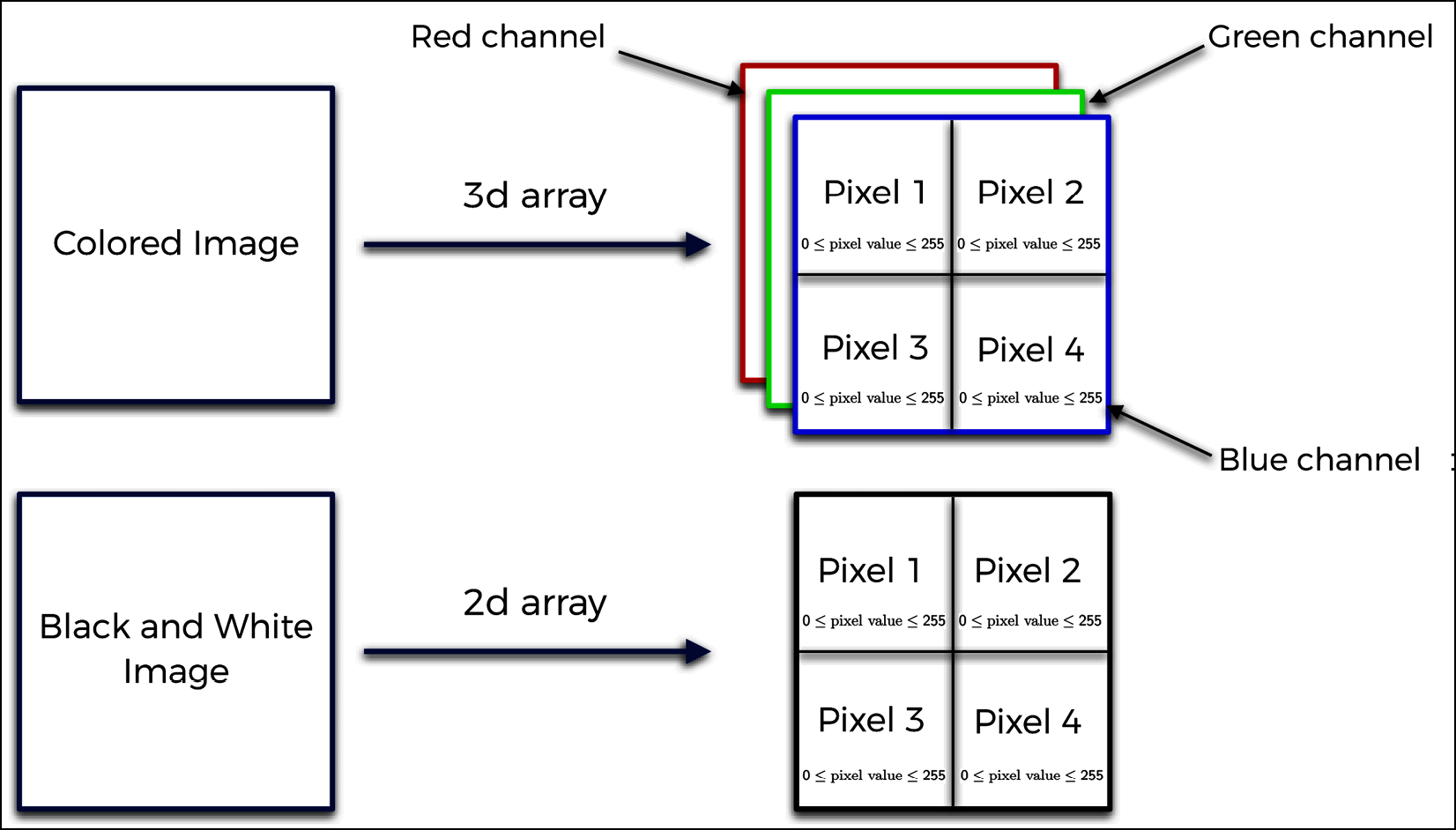
Figure 4...