



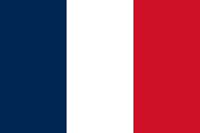


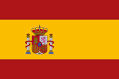



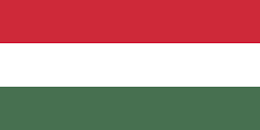

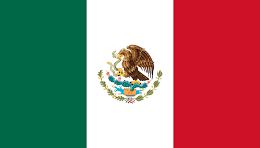


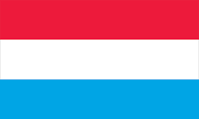



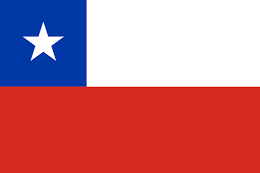


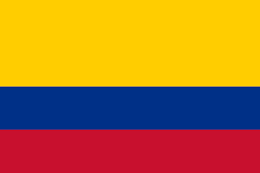
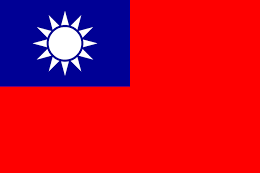


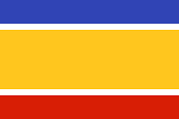
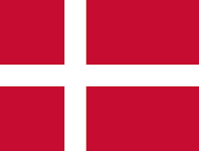
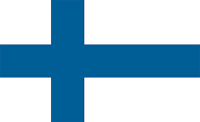



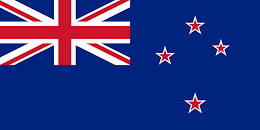





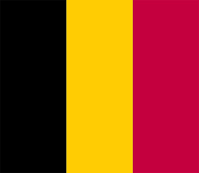

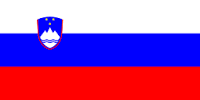




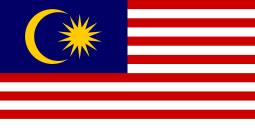


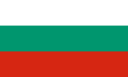



Edge services and edge computing have been in talks since at least the 90s.
When Edge computing is extended to the cloud it can be managed and consumed as if it were local infrastructure. The logic is simple. It’s the same as how humans find it hard to interact with infrastructure that is too far away.
Edge Analytics is the exciting area of data analytics that is gaining a lot of attention these days. While traditional analytics, answer questions like what happened, why it happened, what is likely to happen and options on what you should do about it Edge analytics is data analytics in real time. It deals with the operations performed on data at the edge of a network either at or close to a sensor, a network switch or some other connected device.
This saves time and overhead issues as well as latency problems. As they rightly say, Time is money! Now imagine using AI to facilitate edge analytics.
When Edge computing is extended to the cloud it can be managed and consumed as if it were local infrastructure. The logic is simple. It’s the same as how humans find it hard to interact with infrastructure that is too far away.
Smart Applications these rely on sending tons of information to the cloud. Data can be compromised in such situations. As such, security and privacy challenges may arise. Application developers will have to consider whether the bulk of information sent to the cloud contains personally identifiable information (PII) and whether storing it is in breach of privacy laws. They’ll also have to take the necessary measures to secure the information they store and prevent it from being stolen, or accessed or shared illegally. Now that is a lot of work!
Enter “Intelligent Edge” computing used to save the day! Edge computing by itself will not replace the power of the cloud. It can, however, reduce cloud payloads drastically when used in collaboration with machine learning. transform the AI’s operation model into that of the human brain: perform routine and time-critical decisions at the edge and only refer to the cloud where more intensive computation and historical analysis is needed.
Most mobile apps, IoT devices and other applications that work with AI and machine learning algorithms and applications rely on the processing power of the cloud or on a datacenter situated thousands of miles away. They have little or no intelligence to apply processing at the edge. Even if you show your favorite pet picture to your smart device a thousand times, it’ll still have to look it up in its cloud server in order to recognize if its a dog or a cat for the 1001st time.
OK, who cares if it takes a couple of minutes more for my device to differentiate between a dog and a cat!
Let’s consider a robot surgeon which wants to perform a sensitive operation on a patient. It will need to be able to analyze images and make decisions dozens of times per second. The round trip to the cloud would cause lags that could have severe consequences. God forbid, if there is a cloud outage or poor internet connectivity. To perform this task efficiently, faster and to reduce the back and forth communication involved between the cloud and the device, implementing AI in edge is a good idea.
Now that you are convinced that Intelligent edge or AI powered edge computing does have potential, here are some recent advancements in edge AI and some ways it is being used in the real world.
One of the central design principles of Microsoft’s intelligent edge products and services is to secure data no matter where it is stored. Azure Sphere is one of their intelligent edge solutions to power and protect connected microcontroller unit (MCU)-powered devices. There are 9 billion MCU-powered devices shipping every year, which power everything from household stoves and refrigerators to industrial equipment.
That’s intelligent edge for you on the consumer end of the application spectrum. Let’s look at the industrial application use case next.
To make a mark in the field of industrial internet of things (IIoT), GE Digital is adding features to its Predix platform as a service (PaaS). This will let industrial enterprises run predictive analytics as close as possible to data sources, whether they be pumps, valves, heat exchangers, turbines or even machines on the move.
The main idea behind edge computing is to analyze data in near real-time, optimize network traffic and cut costs. GE Digital has been working to integrate the company's field service management (FSM) software with GE products and third-party tools. For example, artificial intelligence-enabled predictive analytics now integrate the Apache Spark AI engine to improve service time estimates.
New application integration features let service providers launch and share FSM data with third-party mobile applications installed on the same device. Read the whole story on Network World.
Machine intelligence has largely been the domain of computer vision (CV) applications such as object recognition. While artificial intelligence technology is thus far still in its infancy, its benefits for advanced driver assistance systems (ADAS), collaborative robots (cobots), sense-and-avoid drones, and a host of other embedded applications are obvious.
Related to the origins of AI technology is the fact that most, if not all, machine learning frameworks were developed to run on data center infrastructure. As a result, the software and tools required to create CNNs/DNNs for embedded targets have been lacking. In the embedded machine learning sense, this has meant that intricate knowledge of both embedded processing platforms and neural network creation has been a prerequisite for bringing AI to the embedded edge – a luxury most organizations do not have or is extremely time-consuming if they do.
Thanks to embedded silicon vendors, this paradigm is set to shift. Based on the power consumption benchmarks, AI technology is quickly approaching deeply embedded levels.
Read the whole article on embedded computing design to know more about how Intelligent edge is changing our outlook towards embedded systems.
Grid Edge Controllers are intelligent servers, deployed as an interface between the edge nodes and the utility’s core network.
Smart Grid, as we know, is essentially the concept of establishing a two-way communication between distribution infrastructure, consumer and the utility head end using Internet Protocol.
From residential rooftops to solar farms, commercial solar, electric vehicles and wind farms, smart meters are generating a ton of data. This helps utilities to view the amount of energy available and required, allowing their demand response to become more efficient, avoid peaks and reduce costs. This data is first processed in the Grid Edge Controllers that perform local computation and analysis of the data, only sending necessary actionable information over a wireless network to the Utility.
Using Internet of Things devices such as temperature, humidity, pressure, and moisture sensors, alongside internet protocol (IP) cameras and other technologies, oil and gas monitoring operations produce an immense amount of data which provide key insights into the health of their specific systems.
Edge computing allows this data to be analysed, processed, and then delivered to end-users in real-time. This, in turn, enables control centers to access data as it occurs in order to foresee and prevent malfunctions or incidents before they occur.
Self-driving cars and intelligent traffic management systems are already the talk of the town today and the integration of edge AI could be the next big step.
When it comes to autonomous systems, safety is paramount. Any delay, malfunction, or anomaly within autonomous vehicles can prove to be fatal. Calculating a number of parameters at the same time, edge computing and AI enables safe and fast transportation with quick decision making capabilities.
Edge computing is able to analyse and process data on the traffic hardware itself and finds ways to remove unnecessary traffic. This reduces the overall amount of data that needs to be transmitted across a given network and helps to reduce both operating and storage costs.
The intelligent edge will allow humans to simplify multi-faceted processes by replacing the manual process of sorting and identifying complex data, key insights and actionable plans. This forte of technology can help humans gain a competitive edge by having better decision-making, improved ROI, operational efficiency and cost savings.
However, on the flip side, there are also many cons to machine learning based edge computing..
All-in-All even though the concept of incorporating AI into edge computing is exciting, some work does need to be done to get intelligent edge-based solutions l fully set up, functional and running smoothly in production. What’s your take on this digital transformation?
Reinforcement learning model optimizes brain cancer treatment
Tesla is building its own AI hardware for self-driving cars
OpenAI builds reinforcement learning based system giving robots human like dexterity