



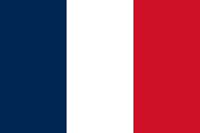


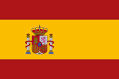



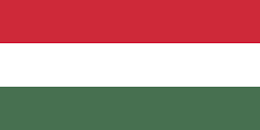

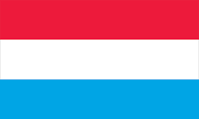





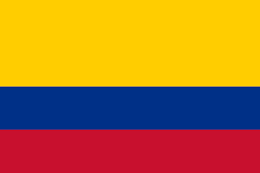
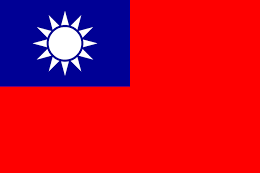
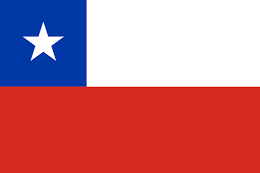



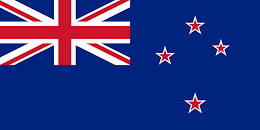
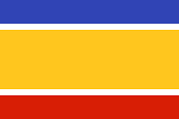
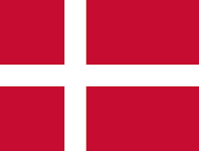
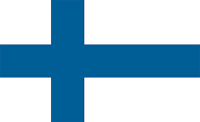







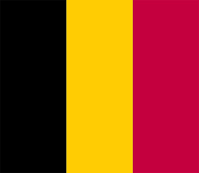

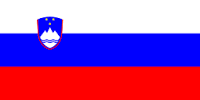





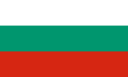


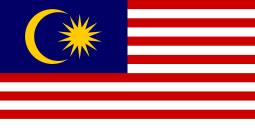



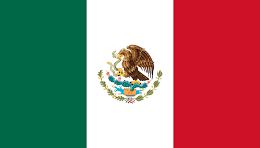

One of the goals for artificial intelligence in biology is the creation of controllable predictive and generative models that can read and generate biology in its native language. Artificial neural networks with proven pattern recognition capabilities, have been utilized in many areas of bioinformatics. Accordingly, research is necessary into methods that can learn intrinsic biological properties directly from protein sequences, which can be transferred to prediction and generation.
Last week, Alexander Rives and Rob Fergus from Dept. of Computer Science, New York University, Siddharth Goyal, Joshua Meier, Demi Guo, Myle Ott, C. Lawrence Zitnick and Jerry Ma from Facebook AI Research team together published a paper titled ‘Biological Structure And Function Emerge From Scaling Unsupervised Learning to 250 Million Protein Sequences’. This paper investigates scaling high-capacity neural networks to extract general and transferable information about proteins from raw sequences.
The next-generation sequencing (NGS) have revolutionized the biological field. It has also helped in performing a wide variety of applications and study biological systems at a detailed level. Recently due to reductions in the cost of this technology, there has been exponential growth in the size of biological sequence datasets. When data is sampled across diverse sequences, it helps in studying predictive and generative techniques for biology using artificial intelligence. In this paper the team has investigated deep learning across evolution at the scale of the largest available protein sequence databases.
Researchers have applied self-supervision to the problem of understanding protein sequences and explore information about representation learning. They have trained a neural network by predicting masked amino acids. For training the neural network, a wide range of datasets containing 250M protein sequences with 86 billion amino acids are used during the research. The resulting model maps raw sequences to representations of biological properties without any prior domain knowledge.
The neural network represents the identity of each amino acid in its input and output embeddings. The space of representations learned from sequences provides biological structure information at many levels, including that of amino acids, proteins, groups of orthologous genes, and species. Information about secondary and tertiary structure is internalized and represented within the network in a generalizable form.
Finally the paper states that it is possible to adapt networks that have been trained on evolutionary data which will give results using only features that have been learned from sequences i.e., without any prior knowledge. It was also observed that the higher capacity models which were trained, were not fit for the 250M sequences, due to insufficient model capacity.
The researchers are certain that using trained network architectures, along with predictive models will help in generating and optimizing new sequences for desired functions. It will also work for sequences that have not been seen before in nature but that are biologically active. They have tried to use unsupervised learning to recover representations that can map multiple levels of biological granularity.
https://twitter.com/soumithchintala/status/1123236593903423490
But the result of the paper does not satisfy the community completely. Some are of the opinion that the paper is incomprehensible and has left some information unarticulated. For example, it is not specified which representation of biological properties does the model map.
A user on Reddit commented that, “Like some of the other ML/AI posts that made it to the top page today, this research too does not give any clear way to reproduce the results. I looked through the pre-print page as well as the full manuscript itself. Without reproducibility and transparency in the code and data, the impact of this research is ultimately limited. No one else can recreate, iterate, and refine the results, nor can anyone rigorously evaluate the methodology used”.
Another user added, “This is cool, but would be significantly cooler if they did some kind of biological follow up. Perhaps getting their model to output an "ideal" sequence for a desired enzymatic function and then swapping that domain into an existing protein lacking the new function”.
Create machine learning pipelines using unsupervised AutoML [Tutorial]
Rigetti develops a new quantum algorithm to supercharge unsupervised Machine Learning
RIP Nils John Nilsson; an AI visionary, inventor of A* algorithm, STRIPS automatic planning system and many more