



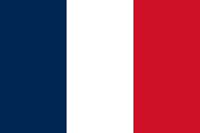


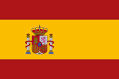



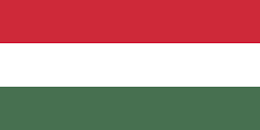

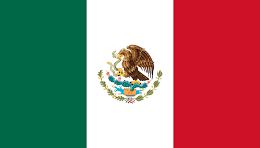


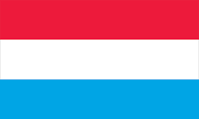



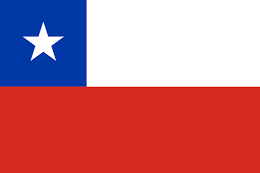


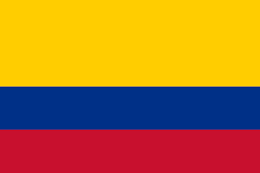
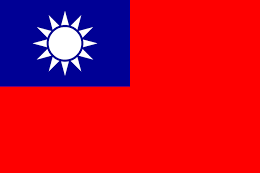


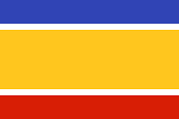
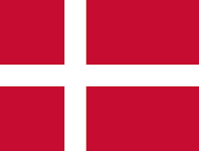
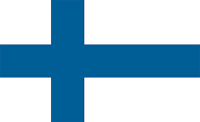



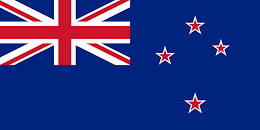





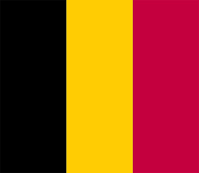

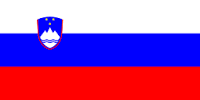




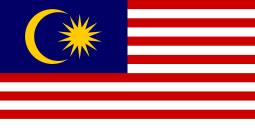


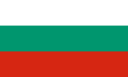



Dive deeper into the world of AI innovation and stay ahead of the AI curve! Subscribe to our AI_Distilled newsletter for the latest insights. Don't miss out – sign up today! 👋 Hello, "If we don't embrace AI, it will move forward without us. Now is the time to harness AI's potential for the betterment of society." - Fei-Fei Li, Computer Scientist and AI Expert. AI is proving to be a real game-changer worldwide, bringing new perspectives to everyday affairs in every field. No wonder Apple is heavily investing in Siri's generative AI enhancement and Microsoft to Provide Legal Protection for AI-Generated Copyright Breaches however, AI currently has massive cooling requirements in data centers which has led to a 34% increase in water consumption in Microsoft data centers. Say hello to the latest edition of our AI_Distilled #17 where we talk about all things LLM, NLP, GPT, and Generative AI! In this edition, we present the latest AI developments from across the world, including NVIDIA TensorRT-LLM enhances Large Language Model inference on H100 GPUs, Meta developing powerful AI system to compete with OpenAI, Google launching Digital Futures Project to support responsible AI, Adept open-sourcing a powerful language model with <10 billion parameters, and Numenta introduces NuPIC, revolutionizing AI efficiency by 100 Times. We know how much you love our curated AI secret knowledge resources. This week, we’re here with some amazing tutorials on building an AWS conversational AI app with AWS Amplify, how to evaluate legal language models with Azure ML PromptFlow, deploying generative AI models on Amazon EKS with a step-by-step guide, Automate It with Zapier and Generative AI and generating realistic textual synthetic data using LLMs. | |
What do you think of this issue and our newsletter? Please consider taking the short survey below to share your thoughts and you will get a free PDF of the “The Applied Artificial Intelligence Workshop” eBook upon completion. | |
Writer’s Credit: Special shout-out to Vidhu Jain for their valuable contribution to this week’s newsletter content! Cheers, Merlyn Shelley Editor-in-Chief, Packt | |
⚡ TechWave: AI/GPT News & AnalysisGoogle Launches Digital Futures Project to Support Responsible AI: Google has initiated the Digital Futures Project, accompanied by a $20 million fund from Google.org to provide grants to global think tanks and academic institutions. This project aims to unite various voices to understand and address the opportunities and challenges presented by AI. It seeks to support researchers, organize discussions, and stimulate debates on public policy solutions for responsible AI development. The fund will encourage independent research on topics like AI's impact on global security, labor, and governance structures. Inaugural grantees include renowned institutions like the Aspen Institute and MIT Work of the Future. Microsoft to Provide Legal Protection for AI-Generated Copyright Breaches: Microsoft has committed to assuming legal responsibility for copyright infringement related to material generated by its AI software used in Word, PowerPoint, and coding tools. The company will cover legal costs for commercial customers who face lawsuits over tools or content produced by AI. This includes services like GitHub Copilot and Microsoft 365 Copilot. The move aims to ease concerns about potential clashes with content owners and make the software more user-friendly. Other tech companies, such as Adobe, have made similar pledges to indemnify users of AI tools. Microsoft's goal is to provide reassurance to paying users amid the growing use of generative AI, which may reproduce copyrighted content. NVIDIA TensorRT-LLM Enhances Large Language Model Inference on H100 GPUs: NVIDIA introduces TensorRT-LLM, a software solution that accelerates and optimizes LLM inference. This open-source software incorporates advancements achieved through collaboration with leading companies. TensorRT-LLM is compatible with Ampere, Lovelace, and Hopper GPUs, aiming to streamline LLM deployment. It offers an accessible Python API for defining and customizing LLM architectures without requiring deep programming knowledge. Performance improvements are demonstrated with real-world datasets, including a 4.6x acceleration for Meta's Llama 2. Additionally, TensorRT-LLM helps reduce total cost of ownership and energy consumption in data centers, making it a valuable tool for the AI community. Meta Developing Powerful AI System to Compete with OpenAI: The Facebook parent company is reportedly working on a new AI system that aims to rival the capabilities of OpenAI's advanced models. The company intends to launch this AI model next year, and it is expected to be significantly more powerful than Meta's current offering, Llama 2, an open-source AI language model. Llama 2 was introduced in July and is distributed through Microsoft's Azure services to compete with OpenAI's ChatGPT and Google's Bard. This upcoming AI system could assist other companies in developing sophisticated text generation and analysis services. Meta plans to commence training on this new AI system in early 2024. Adept Open-Sources a Powerful Language Model with <10 Billion Parameters: Adept announces the open-source release of Persimmon-8B, a highly capable language model with fewer than 10 billion parameters. This model, made available under an Apache license, is designed to empower the AI community for various use cases. Persimmon-8B stands out for its substantial context size, being 4 times larger than LLaMA2 and 8 times more than GPT-3. Despite using only 0.37x the training data of LLaMA2, it competes with its performance. It includes 70k unused embeddings for multimodal extensions and offers unique inference code combining speed and flexibility. Adept expects this release to inspire innovation in the AI community. Apple Invests Heavily in Siri's Generative AI Enhancement: Apple has significantly increased its investment in AI, particularly in developing conversational chatbot features for Siri. The company is reportedly spending millions of dollars daily on AI research and development. CEO Tim Cook expressed a strong interest in generative AI. Apple's AI journey began four years ago when John Giannandrea, head of AI, formed a team to work on LLMs. The Foundational Models team, led by Ruoming Pang, is at the forefront of these efforts, rivaling OpenAI's investments. Apple plans to integrate LLMs into Siri to enhance its capabilities, but the challenge lies in fitting these large models onto devices while maintaining privacy and performance standards. Numenta Introduces NuPIC: Revolutionizing AI Efficiency by 100 Times: Numenta, a company bridging neuroscience and AI, has unveiled NuPIC (Numenta Platform for Intelligent Computing), a groundbreaking solution rooted in 17 years of brain research. Developed by computing pioneers Jeff Hawkins and Donna Dubinsky, NuPIC aims to make AI processing up to 100 times more efficient. Partnering with game startup Gallium Studios, NuPIC enables high-performance LLMs on CPUs, prioritizing user trust and privacy. Unlike GPU-reliant models, NuPIC's CPU focus offers cost savings, flexibility, and control while maintaining high throughput and low latency. AI Development Increases Water Consumption in Microsoft Data Centers by 34%: The development of AI tools like ChatGPT has led to a 34% increase in Microsoft's water consumption, raising concerns in the city of West Des Moines, Iowa, where its data centers are located. Microsoft, along with tech giants like OpenAI and Google, has seen rising demand for AI tools, which comes with significant costs, including increased water usage. Microsoft disclosed a 34% spike in global water consumption from 2021 to 2022, largely attributed to AI research. A study estimates that ChatGPT consumes 500 milliliters of water every time it's prompted. Google also reported a 20% growth in water use, partly due to AI work. Microsoft and OpenAI stated they are working to make AI systems more efficient and environmentally friendly. | |
🔮 Looking for a New Book from Packt’s Expert Community? | |
![]() | |
Automate It with Zapier and Generative AI - By Kelly Goss, Philip Lakin Are you excited to supercharge your work with Gen AI's automation skills? Check out this new guide that shows you how to become a Zapier automation pro, making your work more efficient and productive in no time! It covers planning, configuring workflows, troubleshooting, and advanced automation creation. It emphasizes optimizing workflows to prevent errors and task overload. The book explores new built-in apps, AI integration, and complex multi-step Zaps. Additionally, it provides insights into account management and Zap issue resolution for improved automation skills. | |
🌟 Secret Knowledge: AI/LLM ResourcesUnderstanding Liquid Neural Networks: A Primer on AI Advancements: In this post, you'll learn how liquid neural networks are transforming the AI landscape. These networks, inspired by the human brain, offer a unique and creative approach to problem-solving. They excel in complex tasks such as weather prediction, stock market analysis, and speech recognition. Unlike traditional neural networks, liquid neural networks require significantly fewer neurons, making them ideal for resource-constrained environments like autonomous vehicles. These networks excel in handling continuous data streams but may not be suitable for static data. They also provide better causality handling and interpretability. Navigating Generative AI with FMOps and LLMOps: A Practical Guide: In this informative post, you'll gain valuable insights into the world of generative AI and its operationalization using FMOps and LLMOps principles. The authors delve into the challenges businesses face when integrating generative AI into their operations. You'll explore the fundamental differences between traditional MLOps and these emerging concepts. The post outlines the roles various teams play in this process, from data engineers to data scientists, ML engineers, and product owners. The guide provides a roadmap for businesses looking to embrace generative AI. AI Compiler Quartet: A Breakdown of Cutting-Edge Technologies: Explore Microsoft’s groundbreaking "heavy-metal quartet" of AI compilers: Rammer, Roller, Welder, and Grinder. These compilers address the evolving challenges posed by AI models and hardware. Rammer focuses on optimizing deep neural network (DNN) computations, improving hardware parallel utilization. Roller tackles the challenge of memory partitioning and optimization, enabling faster compilation with good computation efficiency. Welder optimizes memory access, particularly vital as AI models become more memory-intensive. Grinder addresses complex control flow execution in AI computation. These AI compilers collectively offer innovative solutions for parallelism, compilation efficiency, memory, and control flow, shaping the future of AI model optimization and compilation. | |
💡 MasterClass: AI/LLM Tutorials
Exploring IoT Data Simulation with ChatGPT and MQTTX: In this comprehensive guide, you'll learn how to harness the power of AI, specifically ChatGPT, and the MQTT client tool, MQTTX, to simulate and generate authentic IoT data streams. Discover why simulating IoT data is crucial for system verification, customer experience enhancement, performance assessment, and rapid prototype design. The article dives into the integration of ChatGPT and MQTTX, introducing the "Candidate Memory Bus" to streamline data testing. Follow the step-by-step guide to create simulation scripts with ChatGPT and efficiently simulate data transmission with MQTTX. Revolutionizing Real-time Inference: SageMaker Unveils Streaming Support for Generative AI: Amazon SageMaker now offers real-time response streaming, transforming generative AI applications. This new feature enables continuous response streaming to clients, reducing time-to-first-byte and enhancing interactive experiences for chatbots, virtual assistants, and music generators. The post guides you through building a streaming web application using SageMaker real-time endpoints for interactive chat use cases. It showcases deployment options with AWS Large Model Inference (LMI) and Hugging Face Text Generation Inference (TGI) containers, providing a seamless, engaging conversation experience for users. Implementing Effective Guardrails for Large Language Models: Guardrails are crucial for maintaining trust in LLM applications as they ensure compliance with defined principles. This guide presents two open-source tools for implementing LLM guardrails: Guardrails AI and NVIDIA NeMo-Guardrails. Guardrails AI offers Python-based validation of LLM responses, using the RAIL specification. It enables developers to define output criteria and corrective actions, with step-by-step instructions for implementation. NVIDIA NeMo-Guardrails introduces Colang, a modeling language for flexible conversational workflows. The guide explains its syntax elements and event-driven design. Comparing the two, Guardrails AI suits simple tasks, while NeMo-Guardrails excels in defining advanced conversational guidelines. | |
🚀 HackHub: Trending AI Tools
cabralpinto/modular-diffusion: Python library for crafting and training personalized Diffusion Models with PyTorch. cofactoryai/textbase: Simplified Python chatbot development using NLP and ML with Textbase's on_message function in main.py. microsoft/BatteryML: Open-source ML tool for battery analysis, aiding researchers in understanding electrochemical processes and predicting battery degradation. facebookresearch/co-tracker: Swift transformer-based video tracker with Optical Flow, pixel-level tracking, grid sampling, and manual point selection. explodinggradients/ragas: Framework evaluates Retrieval Augmented Generation pipelines, enhancing LLM context with external data using research-based tools. |