ML versus traditional software
Before I started working in the field of AI/ML, I spent many years building computer software platforms for large financial services institutions. Some of the business problems I worked on had complex rules, such as identifying companies for comparable analysis for investment banking deals or creating a master database for all the different companies’ identifiers from the different data providers. We had to implement hardcoded rules in database-stored procedures and application server backends to solve these problems. We often debated if certain rules made sense or not for the business problems we tried to solve.
As rules changed, we had to reimplement the rules and make sure the changes did not break anything. To test for new releases or changes, we often replied to human experts to exhaustively test and validate all the business logic implemented before the production release. It was a very time-consuming and error-prone process and required a significant amount of engineering, testing against the documented specification, and rigorous change management for deployment every time new rules were introduced, or existing rules needed to be changed. We often replied to users to report business logic issues in production, and when an issue was reported in production, we sometimes had to open up the source code to troubleshoot or explain the logic of how it worked. I remember I often asked myself if there were better ways to do this.
After I started working in the field of AI/ML, I started to solve many similar challenges using ML techniques. With ML, I did not need to come up with complex rules that often require deep data and domain expertise to create or maintain the complex rules for decision making. Instead, I focused on collecting high-quality data and used ML algorithms to learn the rules and patterns from the data directly. This new approach eliminated many of the challenging aspects of creating new rules (for example, a deep domain expertise requirement, or avoiding human bias) and maintaining existing rules. To validate the model before the production release, we could examine model performance metrics such as accuracy. While it still required data science expertise to interpret the model metrics against the nature of the business problems and dataset, it did not require exhaustive manual testing of all the different scenarios. When a model was deployed into production, we would monitor if the model performed as expected by monitoring any significant changes in production data versus the data we have collected for model training. We would collect new unseen data and labels for production data and test the model performance periodically to ensure that its predictive accuracy remains robust when faced with new, previously unseen production data. To explain why a model made a decision the way it did, we did not need to open up the source code to re-examine the hardcoded logic. Instead, we would rely on ML techniques to help explain the relative importance of different input features to understand what factors were most influential in the decision-making by the ML models.
The following figure shows a graphical view of the process differences between developing a piece of software and training an ML model:
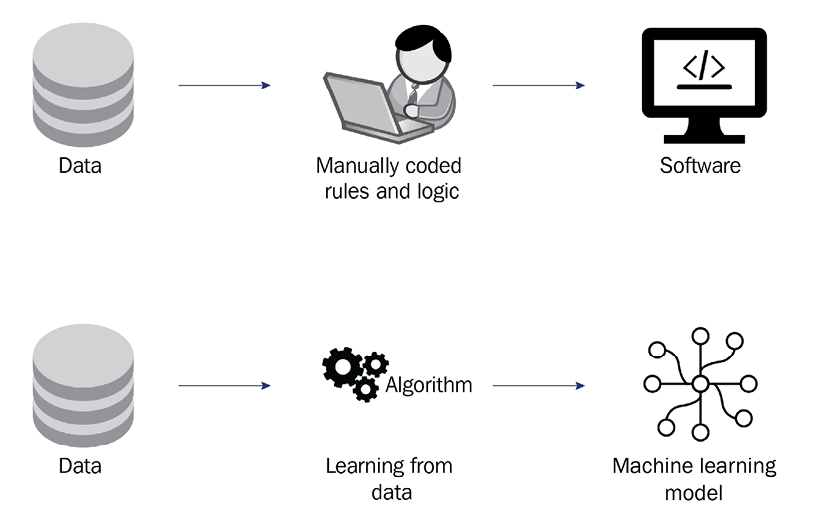
Figure 1.1: ML and computer software
Now that you know the difference between ML and traditional software, it is time to dive deep into understanding the different stages in an ML lifecycle.