Virtual environments
The Python ecosystem offers many methods of installing and managing packages. You can simply download and extract code to your project directory, use the package manager from your operating system, or use a tool such as pip
to install a package. To make sure your packages don’t collide, it is recommended that you use a virtual environment. A virtual environment is a lightweight Python installation with its own package directories and a Python binary copied (or linked) from the binary used to create the environment.
Why virtual environments are a good idea
It might seem like a hassle to create a virtual environment for every Python project, but it offers enough advantages to do so. More importantly, there are several reasons why installing packages globally using pip
is a really bad idea:
- Installing packages globally usually requires elevated privileges (such as
sudo
,root
, oradministrator
), which is a huge security risk. When executingpip install <package>
, thesetup.py
of that package is executed as the user that executed thepip install
command. That means that if the package contains malware, it now has superuser privileges to do whatever it wants. Don’t forget that anyone can upload a package to PyPI (pypi.org) without any vetting. As you will see later in this book, it only takes a couple of minutes for anyone to create and upload a package. - Depending on how you installed Python, it can mess with the existing packages that are installed by your package manager. On an Ubuntu Linux system, that means you could break
pip
or evenapt
itself because apip install -U <package>
installs and updates both the package and all of the dependencies. - It can break your other projects. Many projects try their best to remain backward compatible, but every
pip install
could pull in new/updated dependencies that could break compatibility with other packages and projects. The Django Web Framework, for example, changes enough between versions that many projects using Django will need several changes after an upgrade to the latest release. So, when you’re upgrading Django on your system to the latest version and have a project that was written for a previous version, your project will most likely be broken. - It pollutes your list of packages, making it hard to keep track of your project’s dependencies.
In addition to alleviating the issues above, there is a major advantage as well. You can specify the Python version (assuming you have it installed) when creating the virtual environment. This allows you to test and debug your projects in multiple Python versions easily while keeping the exact same package versions beyond that.
Using venv and virtualenv
You are probably already familiar with virtualenv
, a library used to create a virtual environment for your Python installation. What you might not know is the venv
command, which has been included with Python since version 3.3 and can be used as a drop-in replacement for virtualenv
in most cases. To keep things simple, I recommend creating a directory where you keep all of your environments. Some people opt for an env
, .venv
, or venv
directory within the project, but I advise against that for several reasons:
- Your project files are important, so you probably want to back them up as often as possible. By keeping the bulky environment with all of the installed packages outside of your backups, your backups become faster and lighter.
- Your project directory stays portable. You can even keep it on a remote drive or flash drive without having to worry that the virtual environment will only work on a single system.
- It prevents you from accidentally adding the virtual environment files to your source control system.
If you do decide to keep your virtual environment inside your project directory, make sure that you add that directory to your .gitignore
file (or similar) for your version control system. And if you want to keep your backups faster and lighter, exclude it from the backups. With correct dependency tracking, the virtual environment should be easy enough to rebuild.
Creating a venv
Creating a venv
is a reasonably simple process, but it varies slightly according to the operating system being used.
The following examples use the virtualenv
module directly, but for ease I recommend using poetry
instead, which is covered later in this chapter. This module will automatically create a virtual environment for you when you first use it. Before you make the step up to poetry
, however, it is important to understand how virtual environments work.
Since Python 3.6, the pyvenv
command has been deprecated in favor of python -m venv
.
In the case of Ubuntu, the python3-venv
package has to be installed through apt
because the Ubuntu developers have mutilated the default Python installation by not including ensurepip
.
For Linux/Unix/OS X, using zsh
or bash
as a shell, it is:
$ python3 -m venv envs/your_env
$ source envs/your_env/bin/activate
(your_env) $
And for Windows cmd.exe
(assuming python.exe
is in your PATH
), it is:
C:\Users\wolph>python.exe -m venv envs\your_env
C:\Users\wolph>envs\your_env\Scripts\activate.bat
(your_env) C:\Users\wolph>
PowerShell is also supported and can be used in a similar fashion:
PS C:\Users\wolph>python.exe -m venv envs\your_env
PS C:\Users\wolph> envs\your_env\Scripts\Activate.ps1
(your_env) PS C:\Users\wolph>
The first command creates the environment and the second activates the environment. After activating the environment, commands such as python
and pip
use the environment-specific versions, so pip
install
only installs within your virtual environment. A useful side effect of activating the environment is the prefix with the name of your environment, which is (your_env)
in this case.
Note that we are not using sudo
or other methods of elevating privileges. Elevating privileges is both unnecessary and a potential security risk, as explained in the Why virtual environments are a good idea section.
Using virtualenv
instead of venv
is as simple as replacing the following command:
$ python3 -m venv envs/your_env
with this one:
$ virtualenv envs/your_env
An additional advantage of using virtualenv
instead of venv
, in that case, is that you can specify the Python interpreter:
$ virtualenv -p python3.8 envs/your_env
Whereas with the venv
command, it uses the currently running Python installation, so you need to change it through the following invocation:
$ python3.8 -m venv envs/your_env
Activating a venv/virtualenv
Every time you get back to your project after closing the shell, you need to reactivate the environment. The activation of a virtual environment consists of:
- Modifying your
PATH
environment variable to useenvs\your_env\Script
orenvs/your_env/bin
for Windows or Linux/Unix, respectively - Modifying your prompt so that instead of
$
, you see(your_env) $
, indicating that you are working in a virtual environment
In the case of poetry
, you can use the poetry shell
command to create a new shell with the activated environment.
While you can easily modify those manually, an easier method is to run the activate
script that was generated when creating the virtual environment.
For Linux/Unix with zsh
or bash
as the shell, it is:
$ source envs/your_env/bin/activate
(your_env) $
For Windows using cmd.exe
, it is:
C:\Users\wolph>envs\your_env\Scripts\activate.bat
(your_env) C:\Users\wolph>
For Windows using PowerShell, it is:
PS C:\Users\wolph> envs\your_env\Scripts\Activate.ps1
(your_env) PS C:\Users\wolph>
By default, the PowerShell permissions might be too restrictive to allow this. You can change this policy for the current PowerShell session by executing:
Set-ExecutionPolicy Unrestricted -Scope Process
If you wish to permanently change it for every PowerShell session for the current user, execute:
Set-ExecutionPolicy Unrestricted -Scope CurrentUser
Different shells, such as fish
and csh
, are also supported by using the activate.fish
and activate.csh
scripts, respectively.
When not using an interactive shell (with a cron job, for example), you can still use the environment by using the Python interpreter in the bin
or scripts
directory for Linux/Unix or Windows, respectively. Instead of running python script.py
or /usr/bin/python script.py
, you can use:
/home/wolph/envs/your_env/bin/python script.py
Note that commands installed through pip
(and pip
itself) can be run in a similar fashion:
/home/wolph/envs/your_env/bin/pip
Installing packages
Installing packages within your virtual environment can be done using pip
as normal:
$ pip3 install <package>
The great advantage comes when looking at the list of installed packages:
$ pip3 freeze
Because our environment is isolated from the system, we only see the packages and dependencies that we have explicitly installed.
Fully isolating the virtual environment from the system Python packages can be a downside in some cases. It takes up more disk space and the package might not be in sync with the C/C++ libraries on the system. The PostgreSQL database server, for example, is often used together with the psycopg2
package. While binaries are available for most platforms and building the package from the source is fairly easy, it can sometimes be more convenient to use the package that is bundled with your system. That way, you are certain that the package is compatible with both the installed Python and PostgreSQL versions.
To mix your virtual environment with system packages, you can use the --system-site-packages
flag when creating the environment:
$ python3 -m venv --system-site-packages envs/your_env
When enabling this flag, the environment will have the system Python environment sys.path
appended to your virtual environment’s sys.path
, effectively providing the system packages as a fallback when an import
from the virtual environment fails.
Explicitly installing or updating a package within your virtual environment will effectively hide the system package from within your virtual environment. Uninstalling the package from your virtual environment will make it reappear.
As you might suspect, this also affects the results of pip freeze
. Luckily, pip freeze
can be told to only list the packages local to the virtual environment, which excludes the system packages:
$ pip3 freeze --local
Later in this chapter, we will discuss pipenv,
which transparently handles the creation of the virtual environment for you.
Using pyenv
The pyenv
library makes it really easy to quickly install and switch between multiple Python versions. A common issue with many Linux and Unix systems is that the package managers opt for stability over recency. In most cases, this is definitely an advantage, but if you are running a project that requires the latest and greatest Python version, or a really old version, it requires you to compile and install it manually. The pyenv
package makes this process really easy for you but does still require the compiler to be installed.
A nice addition to pyenv
for testing purposes is the tox
library. This library allows you to run your tests on a whole list of Python versions simultaneously. The usage of tox
is covered in Chapter 10, Testing and Logging – Preparing for Bugs.
To install pyenv
, I recommend visiting the pyenv
project page, since it depends highly on your operating system and operating system version. For Linux/Unix, you can use the regular pyenv
installation manual or the pyenv-installer
(https://github.com/pyenv/pyenv-installer) one-liner, if you deem it safe enough:
$ curl https://pyenv.run | bash
Make sure that you follow the instructions given by the installer. To ensure pyenv
works properly, you will need to modify your .zshrc
or .bashrc
.
Windows does not support pyenv
natively (outside of Windows Subsystem for Linux) but has a pyenv
fork available: https://github.com/pyenv-win/pyenv-win#installation
After installing pyenv
, you can view the list of supported Python versions using:
$ pyenv install --list
The list is rather long, but can be shortened with grep
on Linux/Unix:
$ pyenv install --list | grep 3.10
3.10.0
3.10-dev
...
Once you’ve found the version you like, you can install it through the install
command:
$ pyenv install 3.10-dev
Cloning https://github.com/python/cpython...
Installing Python-3.10-dev...
Installed Python-3.10-dev to /home/wolph/.pyenv/versions/3.10-dev
The pyenv install
command takes an optional --debug
parameter, which builds a debug version of Python that makes debugging C/C++ extensions possible using a debugger such as gdb
.
Once the Python version has been built, you can activate it globally, but you can also use the pyenv-virtualenv
plugin (https://github.com/pyenv/pyenv-virtualenv) to create a virtualenv
for your newly created Python environment:
$ pyenv virtualenv 3.10-dev your_pyenv
you can see in the preceding example, as opposed to the venv
and virtualenv
commands, pyenv virtualenv
automatically creates the environment in the ~/.pyenv/versions/<version>/envs/
directory so you’re not allowed to fully specify your own path. You can change the base path (~/.pyenv/
) through the PYENV_ROOT
environment variable, however. Activating the environment using the activate
script in the environment directory is still possible, but more complicated than it needs to be since there’s an easy shortcut:
$ pyenv activate your_pyenv
Now that the environment is activated, you can run environment-specific commands, such as pip
, and they will only modify your environment.
Using Anaconda
Anaconda is a distribution that supports both the Python and R programming languages. It is much more than simply a virtual environment manager, though; it’s a whole different Python distribution with its own virtual environment system and even a completely different package system. In addition to supporting PyPI, it also supports conda-forge
, which features a very impressive number of packages focused on scientific computing.
For the end user, the most important difference is that packages are installed through the conda
command instead of pip
. This brings a much more advanced dependency check when installing packages. Whereas pip
will simply install a package and all of its dependencies without regard for other installed packages, conda
will look at all of the installed packages and make sure it won’t install a version that is not supported by the installed packages.
The conda
package manager is not alone in smart dependency checking. The pipenv
package manager (discussed later in this chapter) does something similar.
Getting started with Anaconda Navigator
Installing Anaconda is quite easy on all common platforms. For Windows, OS X, and Linux, you can go to the Anaconda site and download the (graphical) installer: https://www.anaconda.com/products/distribution#Downloads
Once it’s installed, the easiest way to continue is by launching Anaconda Navigator, which should look something like this:
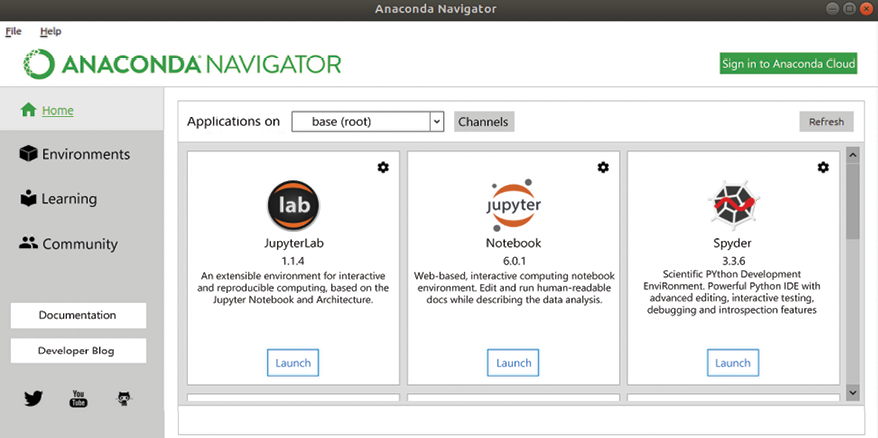
Figure 1.1: Anaconda Navigator – Home
Creating an environment and installing packages is pretty straightforward as well:
- Click on the Environments button on the left.
- Click on the Create button below.
- Enter your name and Python version.
- Click on Create to create your environment and wait a bit until Anaconda is done:
Figure 1.2: Anaconda Navigator – Creating an environment
Once Anaconda has finished creating your environment, you should see a list of installed packages. Installing packages can be done by changing the filter of the package list from Installed to All, marking the checkbox near the packages you want to install, and applying the changes.
While creating an environment, Anaconda Navigator shows you where the environment will be created.
Getting started with conda
While Anaconda Navigator is a really nice tool to use to get an overview, being able to run your code from the command line can be convenient too. With the conda
command, that is luckily very easy.
First, you need to open the conda
shell. You can do this from Anaconda Navigator if you wish, but you can also run it straightaway. On Windows, you can open Anaconda Prompt or Anaconda PowerShell Prompt from the start menu. On Linux and OS X, the most convenient method is to initialize the shell integration. For zsh, you can use:
$ conda init zsh
For other shells, the process is similar. Note that this process modifies your shell configuration to automatically activate the base
environment every time you open a shell. This can be disabled with a simple configuration option:
$ conda config --set auto_activate_base false
If automatic activation is not enabled, you will need to run the activate
command to get back into the conda base
environment:
$ conda activate
(base) $
If, instead of the conda base
environment, you wish to activate the environment you created earlier, you need to specify the name:
$ conda activate conda_env
(conda_env) $
If you have not created the environment yet, you can do so using the command line as well:
$ conda create --name conda_env
Collecting package metadata (current_repodata.json): done
Solving environment: done
...
Proceed ([y]/n)? y
Preparing transaction: done
Verifying transaction: done
Executing transaction: done
...
To list the available environments, you can use the conda info
command:
$ conda info --envs
# conda environments
#
base * /usr/local/anaconda3
conda_env /usr/local/anaconda3/envs/conda_env
Installing conda packages
Now it’s time to install a package. For conda
packages, you can simply use the conda install
command. For example, to install the progressbar2
package that I maintain, use:
(conda_env) $ conda install progressbar2
Collecting package metadata (current_repodata.json): done
Solving environment: done
## Package Plan ##
environment location: /usr/local/anaconda3/envs/conda_env
added / updated specs:
- progressbar2
The following packages will be downloaded:
...
The following NEW packages will be INSTALLED:
...
Proceed ([y]/n)? y
Downloading and Extracting Packages
...
Now you can run Python and see that the package has been installed and is working properly:
(conda_env) $ python
Python 3.8.0 (default, Nov 6 2019, 15:49:01)
[Clang 4.0.1 (tags/RELEASE_401/final)] :: Anaconda, Inc. on darwin
Type "help", "copyright", "credits" or "license" for more information.
>>> import progressbar
>>> for _ in progressbar.progressbar(range(5)): pass
...
100% (5 of 5) |##############################| Elapsed Time: 0:00:00 Time: 0:00:00
Another way to verify whether the package has been installed is by running the conda
list
command, which lists the installed packages similarly to pip list
:
(conda_env) $ conda list
# packages in environment at /usr/local/anaconda3/envs/conda_env:
#
# Name Version Build Channel
...
Installing PyPI packages
With PyPI packages, we have two options within the Anaconda distribution. The most obvious is using pip
, but this has the downside of partially circumventing the conda
dependency checker. While conda install
will take the packages installed through PyPI into consideration, the pip
command might upgrade packages undesirably. This behavior can be improved by enabling the conda
/pip
interoperability setting, but this seriously impacts the performance of conda
commands:
$ conda config --set pip_interop_enabled True
Depending on how important fixed versions or conda
performance is for you, you can also opt for converting the package to a conda
package:
(conda_env) $ conda skeleton pypi progressbar2
Warning, the following versions were found for progressbar2
...
Use --version to specify a different version.
...
## Package Plan ##
...
The following NEW packages will be INSTALLED:
...
INFO:conda_build.config:--dirty flag and --keep-old-work not specified. Removing build/test folder after successful build/test.
Now that we have a package, we can modify the files if needed, but using the automatically generated files works most of the time. All that is left now is to build and install the package:
(conda_env) $ conda build progressbar2
...
(conda_env) $ conda install --use-local progressbar2
Collecting package metadata (current_repodata.json): done
Solving environment: done
...
And now we are done! The package has been installed through conda
instead of pip
.
Sharing your environment
When collaborating with others, it is essential to have environments that are as similar as possible to avoid debugging local issues. With pip
, we can simply create a requirements file by using pip freeze
, but that will not include the conda
packages. With conda
, there’s actually an even better solution, which stores not only the dependencies and versions but also the installation channels, environment name, and environment location:
(conda_env) $ conda env export –file environment.yml
(conda_env) $ cat environment.yml
name: conda_env
channels:
- defaults
dependencies:
...
prefix: /usr/local/anaconda3/envs/conda_env
Installing the packages from that environment file can be done while creating the environment:
$ conda env create --name conda_env –file environment.yml
Or they can be added to an existing environment:
(conda_env) $ conda env update --file environment.yml
Collecting package metadata (repodata.json): done
...