



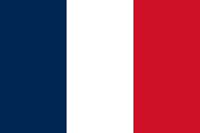


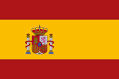



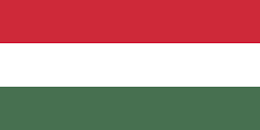

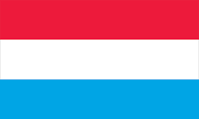





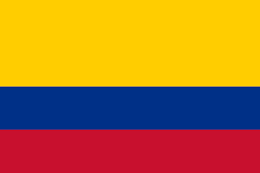
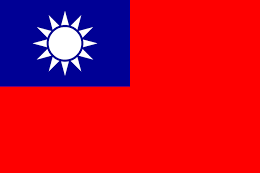
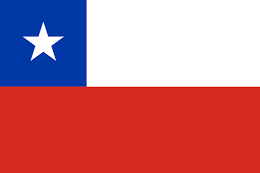



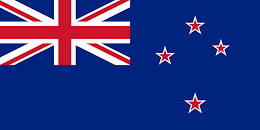
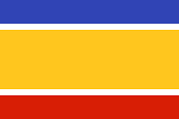
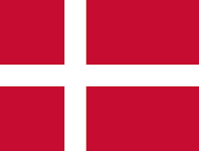
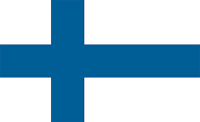







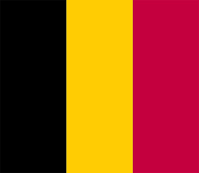

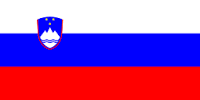





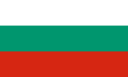


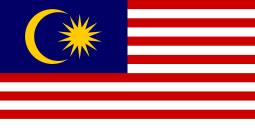



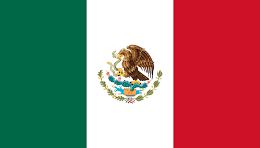

By AI Trends Staff
Looking inside and outside, AI is being applied to the self-diagnosis of automobiles and to the connection of vehicles to traffic infrastructure.
A data scientist at BMW Group in Munich, while working on his PhD, created a system for self-diagnosis called the Automated Damage Assessment Service, according to an account in Mirage. Milan Koch was completing his studies at the Leiden Institute of Advanced Computer Science in the Netherlands when he got the idea. “It should be a nice experience for customers,” he stated.
The system gathers data over time from sensors in different parts of the car. “From scratch, we have developed a service idea that is about detecting damaged parts from low speed accidents,” Koch stated. “The car itself is able to detect the parts that are broken and can estimate the costs and the time of the repair.”
Koch developed and compared different multivariate time series methods, based on machine learning, deep learning and also state-of-the-art automated machine learning (AutoML) models. He tested different levels of complexity to find the best way to solve the time series problems. Two of the AutoML methods and his hand-crafted machine learning pipeline showed the best results.
The system may have application to other multivariate time series problems, where multiple time-dependent variables must be considered, outside the automotive field. Koch collaborated with researchers from the Leiden University Medical Center (LUMC) to use his hand-crafted pipeline to analyze Electroencephalography (EEG) data.
Koch stated, ‘We predicted the cognition of patients based on EEG data, because an accurate assessment of cognitive function is required during the screening process for Deep Brain Stimulation (DBS) surgery. Patients with advanced cognitive deterioration are considered suboptimal candidates for DBS as cognitive function may deteriorate after surgery. However, cognitive function is sometimes difficult to assess accurately, and analysis of EEG patterns may provide additional biomarkers. Our machine learning pipeline was well suited to apply to this problem.”
He added, “We developed algorithms for the automotive domain and initially we didn’t have the intention to apply it to the medical domain, but it worked out really well.” His models are now also applied to Electromyography (EMG) data, to distinguish between people with a motor disease and healthy people.
Koch intends to continue his work at BMW Group, where he will focus on customer-oriented services, predictive maintenance applications and optimization of vehicle diagnostics.
DOE Grant to Research Traffic Management Delays Aims to Reduce Emissions
Getting automobiles to talk to the traffic management infrastructure is the goal of research at the University of Tennesse at Chattanooga, which has been awarded $1.89 million from the US Department of Energy to create a new model for traffic intersections that would reduce energy consumption. The UTC Center for Urban Informatics and Progress (CUIP) will leverage its existing “smart corridor” to accommodate the new research. The smart corridor is a 1.25-mile span on a main artery in downtown Chattanooga, used as a test bed for research into smart city development and connected vehicles in a real-world environment.
“This project is a huge opportunity for us,” stated Dr. Mina Sartipi, CUIP Director and principal investigator, in a press release. “Collaborating on a project that is future-oriented, novel, and full of potential is exciting. This work will contribute to the existing body of literature and lead the way for future research.”
UTC is collaborating with the University of Pittsburgh, the Georgia Institute of Technology, the Oak Ridge National Laboratory, and the City of Chattanooga on the project.
In the grant proposal for the DOE, the research team noted that the US transportation sector accounted for more than 69 percent of petroleum consumption, and more than 37 percent of the country’s CO2 emissions. An earlier National Traffic Signal Report Card found that inefficient traffic signals contribute to 295 million vehicle hours of traffic delay, making up to 10 percent of all traffic-related delays.
The project intends to leverage the capabilities of connected vehicles and infrastructures to optimize and manage traffic flow. While adaptive traffic control systems (ATCS) have been in use for a half century to improve mobility and traffic efficiency, they were not designed to address fuel consumption and emissions. Inefficient traffic systems increase idling time and stop-and-go traffic. The National Transportation Operations Coalition has graded the state of the nation’s traffic signals as D+.
“The next step in the evolution [of intelligent transportation systems] is the merging of these systems through AI,” noted Aleksandar Stevanovic, associate professor of civil and environmental engineering at Pitt’s Swanson School of Engineering and director of the Pittsburgh Intelligent Transportation Systems (PITTS) Lab. “Creation of such a system, especially for dense urban corridors and sprawling exurbs, can greatly improve energy and sustainability impacts. This is critical as our transportation portfolio will continue to have a heavy reliance on gasoline-powered vehicles for some time.”
The goal of the three-year project is to develop a dynamic feedback Ecological Automotive Traffic Control System (Eco-ATCS), which reduces fuel consumption and greenhouse gases while maintaining a highly operable and safe transportation environment. The integration of AI will allow additional infrastructure enhancements including emergency vehicle preemption, transit signal priority, and pedestrian safety. The ultimate goal is to reduce corridor-level fuel consumption by 20 percent.
Read the source articles and information in Mirage, and in a press release from the UTC Center for Urban Informatics and Progress.