Building a machine learning pipeline
After cleaning the data and selecting the most important features, the machine learning flow can be summarized into steps, as shown in Figure 7.4:
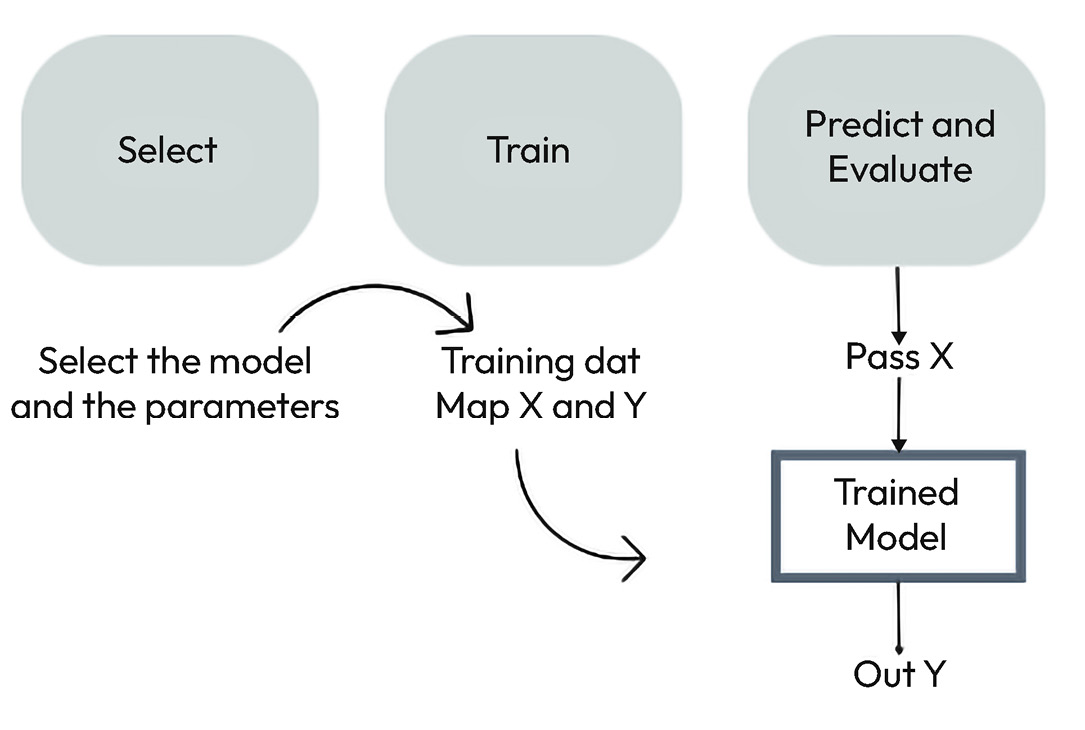
Figure 7.4 – Machine learning pipeline
To carry out this process, we must do the following:
- Select a model and its initial parameters based on the problem and available data.
- Train: First, we must split the data into a training set and a test set. The process of training consists of making the model learn from the data. Each model’s training process can vary in time and computational consumption. To improve the model’s performance, we must employ hyperparameter tuning through techniques such as grid search or random grid search.
- Predict and evaluate: The trained model is then used to predict over the test set, which contains rows of data that have not been seen by the algorithm. If we evaluate the model with the data that we used to train...