We know that in artificial neurons, we multiply the input by weights, add bias to them and apply an activation function to produce the output. Now, we will see how this happens in a neural network setting where neurons are arranged in layers. The number of layers in a network is equal to the number of hidden layers plus the number of output layers. We don't take the input layer into account. Consider a two-layer neural network with one input layer, one hidden layer, and one output layer, as shown in the following diagram:
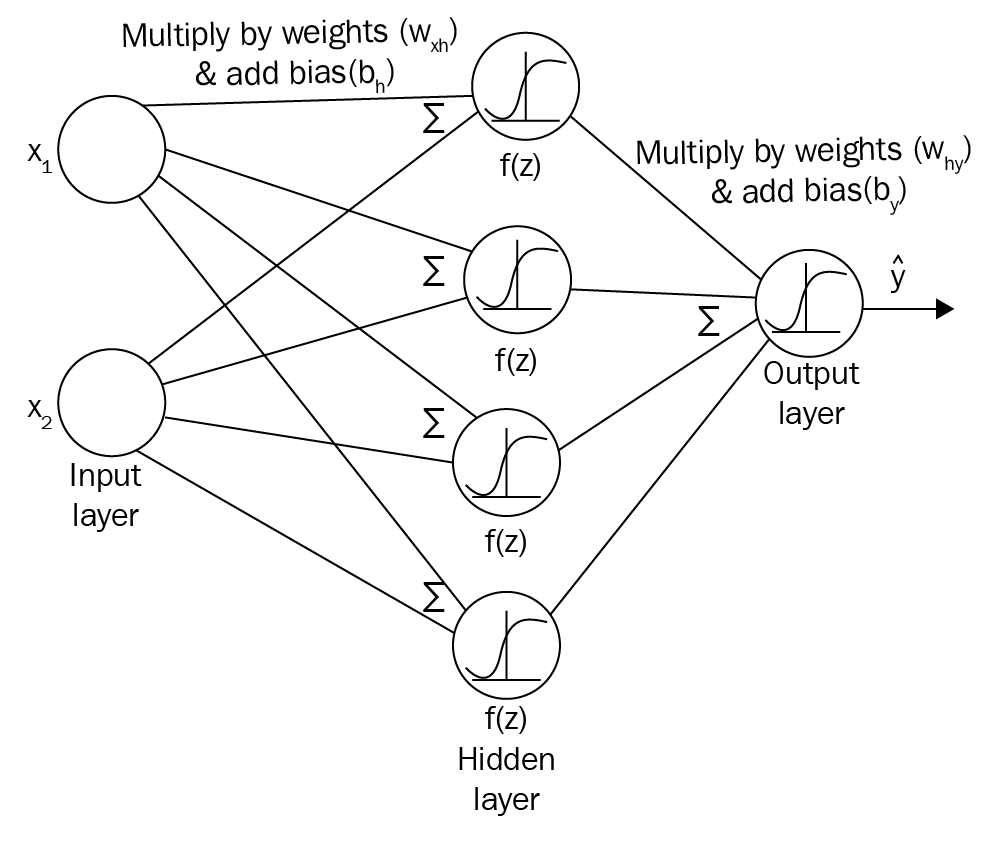
Let's say we have two inputs, x1 and x2, and we have to predict the output y. Since we have two inputs, the number of neurons in the input layer will be two. Now, these inputs will be multiplied by weights and then we add bias and propagate the resultant value to the hidden layer where the activation function will be applied. So...