




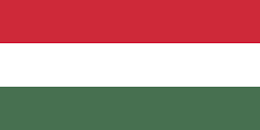

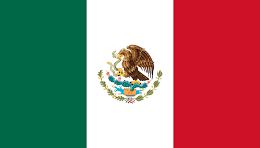


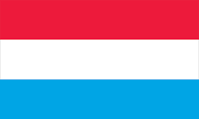



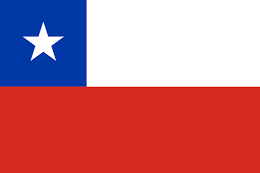



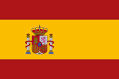


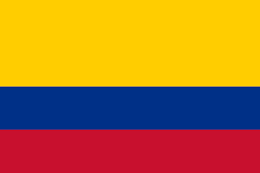
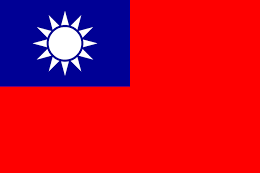


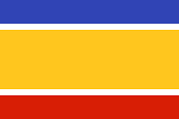
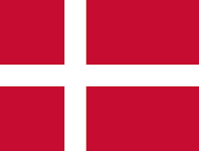
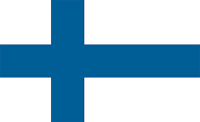



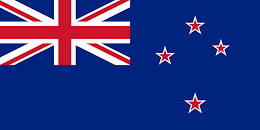


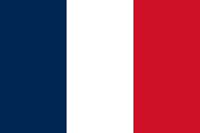



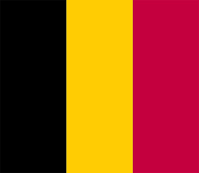

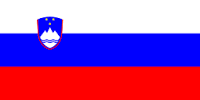




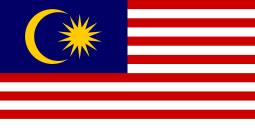


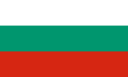




👋 Hello ,
Pichai's AI-fire analogy signifies a transformative era; AI and ML will revolutionize education, medicine, and more, reshaping human progress. OpenAI has begun promoting the use of ChatGPT in education, which shouldn’t really come as a surprise as students the world over have been experimenting with the technology. Get ready to dive into the latest AI developments in this edition, AI_Distilled #16, including Baidu launching Ernie chatbot following Chinese government approval, X's Privacy Policy Reveals Plan to Use Public Data for AI Training, Meta releasing FACET Dataset to evaluate AI model fairness, Google’s new Multislice for scalable AI training on cloud TPUs, and Qualcomm's focus on AI and auto amidst NVIDIA's chip dominance. Watch out also for our handpicked collection of fresh AI, GPT, and LLM-focused secret knowledge and tutorials from around the web covering Liquid Neural Networks, Serverless Machine Learning with Amazon Redshift ML, implementing effective guardrails for LLMs, Navigating Generative AI with FMOps and LLMOps, and using Microsoft’s new AI compiler quartet. | |
What do you think of this issue and our newsletter? Please consider taking the short survey below to share your thoughts and you will get a free PDF of the “The Applied Artificial Intelligence Workshop” eBook upon completion. | |
Writer’s Credit: Special shout-out to Vidhu Jain for their valuable contribution to this week’s newsletter content! Cheers, Merlyn Shelley Editor-in-Chief, Packt | |
⚡ TechWave: AI/GPT News & AnalysisMeta Releases FACET Dataset to Evaluate AI Model Fairness: Meta has launched FACET (FAirness in Computer Vision EvaluaTion), a dataset designed to assess the fairness of AI models used for image and video classification, including identifying people. Comprising 32,000 images with 50,000 labeled individuals, FACET includes demographic and physical attributes, allowing for deep evaluations of biases against various classes. Despite previous concerns about Meta's responsible AI practices, the company claims FACET is more comprehensive than previous bias benchmarks. However, concerns have been raised about the dataset's origins and the compensation of annotators. Meta has also released a web-based dataset explorer tool for FACET. You can read the full paper here. Baidu Launches Ernie Chatbot Following Chinese Government Approval: Chinese tech giant Baidu has unveiled its chatbot, Ernie Bot, after receiving government clearance, along with other AI firms. Ernie Bot is now accessible for download via app stores or Baidu's website. Similar to its rival, ChatGPT, users can engage Ernie Bot for queries, market analysis assistance, marketing slogan ideas, and document summaries. While it's accessible globally, registration requires a Chinese number, and the app is only in Chinese on US Android and iOS stores. Baidu has also introduced a plug-in market for Ernie Bot, which quickly garnered over 1 million users within 19 hours of launch. CEO Robin Li expressed plans for further AI-native apps aimed at exploring generative AI's core abilities. Google Introduces Multislice for Scalable AI Training on Cloud TPUs: Google has unveiled Multislice, a comprehensive large-scale training technology that facilitates straightforward, cost-effective, and nearly linear scaling to tens of thousands of Cloud Tensor Processing Units (TPUs) chips. Traditionally, a training run was restricted to a single slice, which meant a maximum of 3072 TPU v4 chips could be used. With Multislice, training can span multiple slices across pods through data center networking, eliminating these limitations. This innovation offers benefits such as efficient scaling for massive models, enhanced developer productivity, automatic compiler optimizations, and cost-efficiency. It promises to revolutionize AI infrastructure by enabling near-linear scaling for AI supercomputing. OpenAI Promotes Use of ChatGPT in Education: OpenAI is encouraging educators to utilize ChatGPT in classrooms. The company showcased six educators, primarily at the university level, using ChatGPT for various purposes, such as role-playing in debates, aiding translation for English-as-a-second-language students, and fact-checking. Despite some schools banning ChatGPT due to concerns about academic integrity, OpenAI believes it can be a valuable tool in education. However, it emphasizes the importance of maintaining human oversight in the assessment process. X's Privacy Policy Reveals Plan to Use Public Data for AI Training: In an update to its privacy policy, X (formerly Twitter) has informed users that it will now collect biometric data, job histories, and education backgrounds. However, another section of the policy reveals a broader plan: X intends to utilize the data it gathers, along with publicly available information, to train its machine learning and AI models. This revelation has attracted attention, particularly due to the connection with X owner Elon Musk's ambitions in the AI market through his company xAI. Musk confirmed the privacy policy change, emphasizing that only public data, not private messages, would be used for AI training. Qualcomm's Focus on AI and Auto Amidst NVIDIA’s Chip Dominance: As NVIDIA takes the lead as the world's largest fabless chip company, Qualcomm is strategically positioning itself in the AI realm. The company has unveiled in-vehicle generative AI capabilities, expanded into two-wheelers, and forged a partnership with Amazon Web Services. Qualcomm's CEO, Cristiano Amon, believes that generative AI, currently reliant on cloud resources, will transition to local execution, enhancing performance and cost-efficiency. Diversification is also a priority, with Qualcomm's chips powering various smart devices, especially in the automotive sector. Amid uncertainty about its future relationship with Apple, Qualcomm aims to maintain its dominance through innovations in AI and auto tech. InteRecAgent, A Fusion of Language Models and Recommender Systems Introduced: Researchers from the University of Science and Technology of China, in collaboration with Microsoft Research Asia, have introduced InteRecAgent, a cutting-edge framework. This innovation seeks to combine the interactive capabilities of LLMs with the domain-specific precision of traditional recommender systems. Recommender systems play a vital role in various digital domains, but they often struggle with versatile interactions. On the other hand, LLMs excel in conversations but lack domain-specific knowledge. InteRecAgent introduces the "Candidate Memory Bus" to streamline recommendations for LLMs and a "Plan-first Execution with Dynamic Demonstrations" strategy for effective tool interaction. adidas Utilizes AI and NVIDIA RTX for Photorealistic 3D Content: Sportswear giant adidas is partnering with Covision Media, an Italian startup, to revolutionize their online shopping experience. Covision employs AI and NVIDIA RTX technology to develop 3D scanners that allow businesses to create digital twins of their products with stunning realism. This technology can quickly generate 3D scans, capturing textures, colors, and geometry, resulting in lifelike images. adidas is among the first to adopt this technology for automating and scaling e-commerce content production, enhancing their Virtual Try-On feature and replacing traditional product photography with computer-generated content. | |
🔮 Expert Insights from Packt CommunityServerless Machine Learning with Amazon Redshift ML - By Debu Panda, Phil Bates, Bhanu Pittampally, Sumeet Joshi Data analysts and developers use Redshift data with machine learning (ML) models for tasks such as predicting customer behavior. Amazon Redshift ML streamlines this process using familiar SQL commands. A conundrum arises when attempting to decipher these data silos – a formidable challenge that hampers the derivation of meaningful insights essential for organizational clarity. Adding to this complexity, security and performance considerations typically prevent business analysts from accessing data within OLTP systems. The hiccup is that intricate analytical queries weigh down OLTP databases, casting a shadow over their core operations. Here, the solution is the data warehouse, which is a central hub of curated data, used by business analysts and data scientists to make informed decisions by employing the business intelligence and machine learning tools at their disposal. These users make use of Structured Query Language (SQL) to derive insights from this data trove. Here’s where Amazon Redshift Serverless comes in. It’s a key option within Amazon Redshift, a well-managed cloud data warehouse offered by Amazon Web Services (AWS). With cloud-based ease, Amazon Redshift Serverless lets you set up your data storage without infrastructure hassles or cost worries. You pay based on what you use for compute and storage. Amazon Redshift Serverless goes beyond convenience, propelling modern data applications that seamlessly connect to the data lake. The above content is extracted from the book Serverless Machine Learning with Amazon Redshift ML written by Debu Panda, Phil Bates, Bhanu Pittampally, Sumeet Joshi and published in Aug 2023. To get a glimpse of the book's contents, make sure to read the free chapter provided here, or if you want to unlock the full Packt digital library free for 7 days, try signing up now! To learn more, click on the button below. | |
🌟 Secret Knowledge: AI/LLM ResourcesUnderstanding Liquid Neural Networks: A Primer on AI Advancements: In this post, you'll learn how liquid neural networks are transforming the AI landscape. These networks, inspired by the human brain, offer a unique and creative approach to problem-solving. They excel in complex tasks such as weather prediction, stock market analysis, and speech recognition. Unlike traditional neural networks, liquid neural networks require significantly fewer neurons, making them ideal for resource-constrained environments like autonomous vehicles. These networks excel in handling continuous data streams but may not be suitable for static data. They also provide better causality handling and interpretability. Navigating Generative AI with FMOps and LLMOps: A Practical Guide: In this informative post, you'll gain valuable insights into the world of generative AI and its operationalization using FMOps and LLMOps principles. The authors delve into the challenges businesses face when integrating generative AI into their operations. You'll explore the fundamental differences between traditional MLOps and these emerging concepts. The post outlines the roles various teams play in this process, from data engineers to data scientists, ML engineers, and product owners. The guide provides a roadmap for businesses looking to embrace generative AI. AI Compiler Quartet: A Breakdown of Cutting-Edge Technologies: Explore Microsoft’s groundbreaking "heavy-metal quartet" of AI compilers: Rammer, Roller, Welder, and Grinder. These compilers address the evolving challenges posed by AI models and hardware. Rammer focuses on optimizing deep neural network (DNN) computations, improving hardware parallel utilization. Roller tackles the challenge of memory partitioning and optimization, enabling faster compilation with good computation efficiency. Welder optimizes memory access, particularly vital as AI models become more memory-intensive. Grinder addresses complex control flow execution in AI computation. These AI compilers collectively offer innovative solutions for parallelism, compilation efficiency, memory, and control flow, shaping the future of AI model optimization and compilation. | |
💡 MasterClass: AI/LLM Tutorials
Exploring IoT Data Simulation with ChatGPT and MQTTX: In this comprehensive guide, you'll learn how to harness the power of AI, specifically ChatGPT, and the MQTT client tool, MQTTX, to simulate and generate authentic IoT data streams. Discover why simulating IoT data is crucial for system verification, customer experience enhancement, performance assessment, and rapid prototype design. The article dives into the integration of ChatGPT and MQTTX, introducing the "Candidate Memory Bus" to streamline data testing. Follow the step-by-step guide to create simulation scripts with ChatGPT and efficiently simulate data transmission with MQTTX. Revolutionizing Real-time Inference: SageMaker Unveils Streaming Support for Generative AI: Amazon SageMaker now offers real-time response streaming, transforming generative AI applications. This new feature enables continuous response streaming to clients, reducing time-to-first-byte and enhancing interactive experiences for chatbots, virtual assistants, and music generators. The post guides you through building a streaming web application using SageMaker real-time endpoints for interactive chat use cases. It showcases deployment options with AWS Large Model Inference (LMI) and Hugging Face Text Generation Inference (TGI) containers, providing a seamless, engaging conversation experience for users. Implementing Effective Guardrails for Large Language Models: Guardrails are crucial for maintaining trust in LLM applications as they ensure compliance with defined principles. This guide presents two open-source tools for implementing LLM guardrails: Guardrails AI and NVIDIA NeMo-Guardrails. Guardrails AI offers Python-based validation of LLM responses, using the RAIL specification. It enables developers to define output criteria and corrective actions, with step-by-step instructions for implementation. NVIDIA NeMo-Guardrails introduces Colang, a modeling language for flexible conversational workflows. The guide explains its syntax elements and event-driven design. Comparing the two, Guardrails AI suits simple tasks, while NeMo-Guardrails excels in defining advanced conversational guidelines. | |
🚀 HackHub: Trending AI Toolscabralpinto/modular-diffusion: Python library for crafting and training personalized Diffusion Models with PyTorch. cofactoryai/textbase: Simplified Python chatbot development using NLP and ML with Textbase's on_message function in main.py. microsoft/BatteryML: Open-source ML tool for battery analysis, aiding researchers in understanding electrochemical processes and predicting battery degradation. facebookresearch/co-tracker: Swift transformer-based video tracker with Optical Flow, pixel-level tracking, grid sampling, and manual point selection. explodinggradients/ragas: Framework evaluates Retrieval Augmented Generation pipelines, enhancing LLM context with external data using research-based tools. |